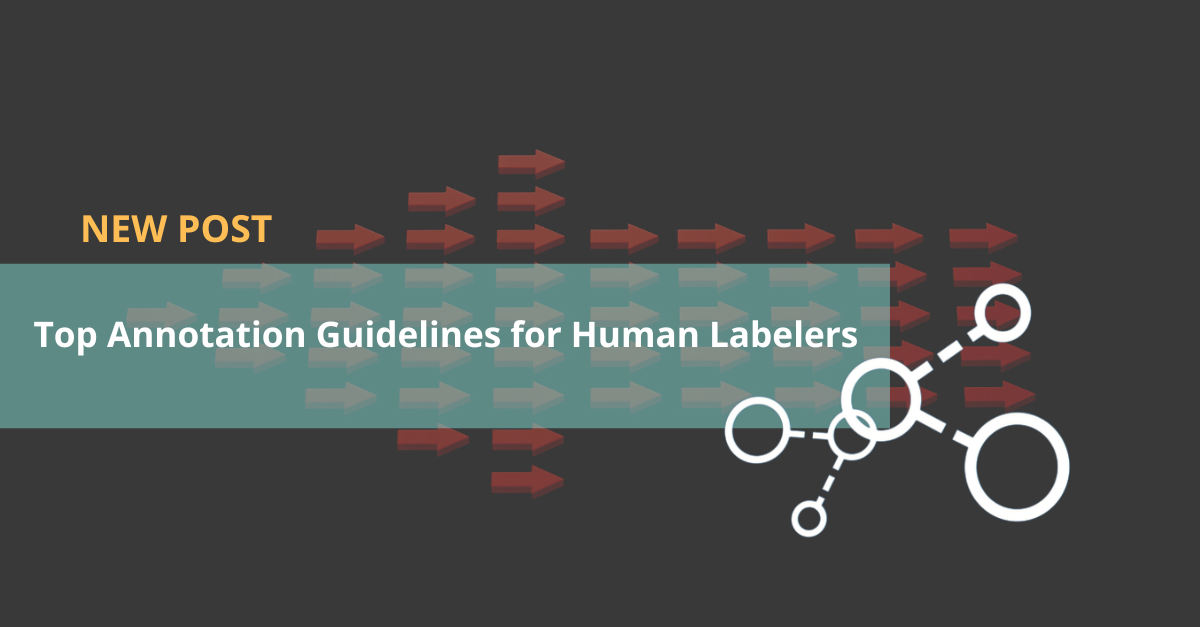
The clarity of annotation guidelines is a key building block to obtain quality data used to train machine learning models. Proper training and annotation guidelines for human labelers are key factors directly determining how well those models will perform. In recent years, machine learning development teams have increasingly recognized the need to optimize data labeling processes.
The production of high-quality data is heavily reliant on the data management practices that machine learning and labeling teams choose to follow. These practices often require manual annotation or the direct involvement of human labelers. At Pangeanic, we would like to share our experiences in speech annotation, image annotation for computer vision systems and text data annotation so you are aware of how we build quality steps throughout the process.
Optimizing a data labeling processes
There are a number of factors to consider when optimizing data labeling processes, including:
-
The type of data being labeled
-
The purpose of the data labeling
-
The level of accuracy required
-
The resources available
Once these factors have been considered, it is possible to develop a data labeling process that is efficient and effective.
Here are some specific tips for optimizing data labeling processes:
-
Use a clear and concise data labeling guidelines. This document should define the data that needs to be labeled, the level of accuracy required, and any other relevant information.
-
Use a well-designed data labeling tool. There are a number of different data labeling tools available, so it is important to choose one that is appropriate for the specific task at hand.
-
Train and monitor your human data labelers. It is important to ensure that your data labelers are properly trained and that they are consistently labeling data to the required level of accuracy.
-
Use quality assurance checks. This is an important step in ensuring that the data labeling process is producing high-quality data.
By following these tips, you can optimize your data labeling processes and ensure that your machine learning models are trained on high-quality data.
Learn more:
The importance of efficient annotation instructions
Inefficient annotation instructions can lead to devalued datasets. This is because data labeling is a repetitive and precise task that requires human input. If the instructions are not clear or thorough, labelers may make mistakes that can impact the quality of the data.
There are a number of factors that can contribute to inefficient annotation instructions. One is the number of labelers involved in the project. If there are many labelers, it can be difficult to ensure that everyone is following the same instructions. Another factor is the expertise of the labelers. If the labelers do not have the necessary expertise, they may not be able to follow the instructions correctly.
The simplest solution to address this issue is to provide comprehensive instructions to labelers. These instructions should be clear, concise, and easy to follow. They should also be tailored to the specific task at hand. In addition, it is important to provide training and support to labelers so that they understand the instructions and can follow them correctly.
By providing comprehensive instructions and training to labelers, you can help to ensure that your data is labeled accurately and efficiently. This will lead to higher-quality data that can be used to train more accurate machine-learning models.
Here are some additional tips for writing effective annotation instructions:
-
Use clear and concise language. Avoid jargon and technical terms that labelers may not understand.
-
Use visuals to support the instructions. This can help labelers to understand the instructions more easily.
-
Break down complex instructions into smaller steps. This will make it easier for labelers to follow the instructions.
-
Provide examples of good and bad annotations. This will help labelers to understand what is expected of them.
-
Test the instructions with a small group of labelers before using them with the full dataset. This will help you to identify any problems with the instructions and make necessary changes.
The Importance of Well-Written Human Annotation Guidelines and Instructions
Well-written instructions are essential for ensuring accurate and consistent data annotation. When instructions are unclear or ambiguous, labelers may make mistakes that can impact the quality of the data.
To write effective annotation instructions, it is important to consider the following:
-
The level of expertise of the labelers. Instructions should be written in a way that is clear and easy to understand for both experienced and inexperienced labelers.
-
The specific task at hand. Instructions should be tailored to the specific task that the labelers are being asked to perform.
-
The type of data being annotated. Instructions should be written in a way that is appropriate for the type of data being annotated.
-
The desired level of accuracy. Instructions should specify the level of accuracy that is expected from the labelers.
It is also important to keep in mind that even the best instructions may not be perfect. As you collect more data and gain more experience with your data labeling process, you may find that you need to make changes to your instructions. Be prepared to revise your instructions as needed to ensure that they are meeting your needs.