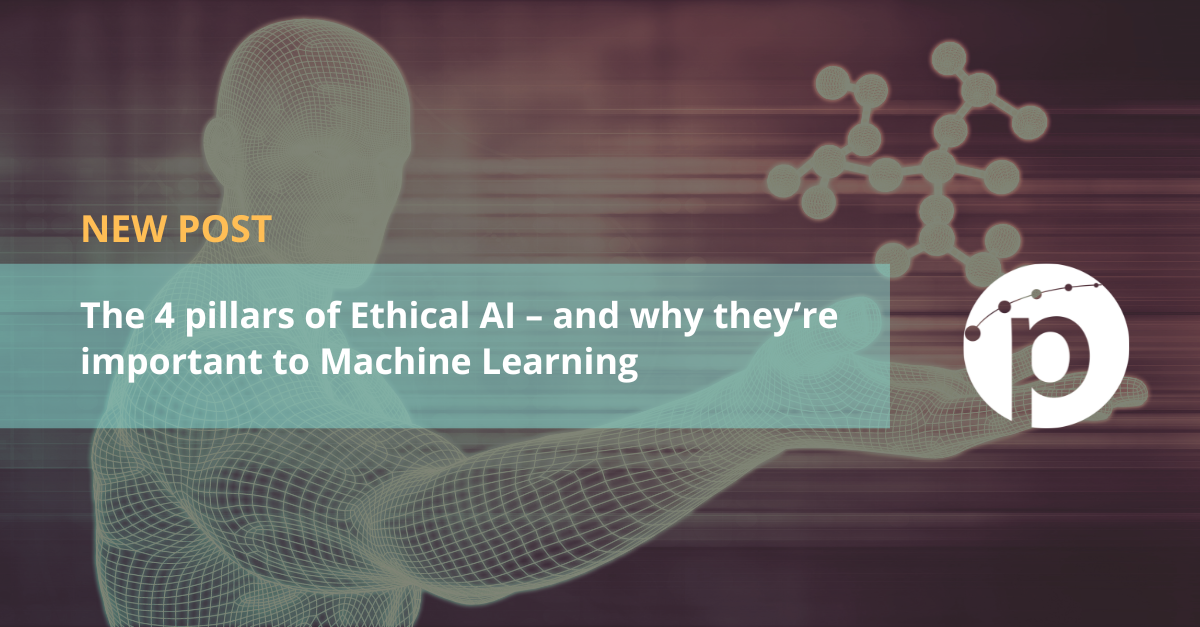
6 min read
14/07/2023
The 4 pillars of Ethical AI – and why they’re important to Machine Learning
Working closely with our clients in the building of Ethical AI systems permeates Pangeanic’s culture and everything we do. But we not only talk the talk but walk the walk. To this end, we want to make public our conscious efforts in data collection, data aggregation and data annotation, building of parallel corpora for ML systems in full compliance with the 4 pillars of Ethical AI. Together with strong multilingual data masking / anonymization technologies, we create data sets and build solutions that based on solid Ethical AI principles.
Content: |
What is Ethical AI?
Ethical AI is a voluntary, worldwide concept that refers to the design, development, inception and deployment of AI systems in a way that respects human rights and shared moral values. It focuses on creating AI systems that are transparent, fair, accountable, and respect privacy. The 4 pillars of Ethical AI are:
-
Transparency: The processes and decisions made by AI systems should be clear, understandable, and explainable. This helps users to understand how a specific decision or output is arrived at by the AI.
-
Fairness: AI systems must avoid biases that can lead to unfair outcomes or discrimination. AI should treat everyone equally and make decisions based on factual data rather than subjective biases.
-
Privacy and Security: AI systems should respect the privacy of users. This means that they should not collect or use personal data without the user's consent. Protecting the privacy and data of individuals is paramount in Ethical AI. It involves using proper data anonymization techniques, ensuring data security, and adhering to all relevant data protection laws and regulations.
-
Accountability: There should be a way to hold those responsible for developing and using AI systems accountable for their actions.
Why is Ethical AI relevant?
Artificial intelligence (AI) advancements are beginning to profoundly affect the way we humans not only interface with machine, but also how we interface with other humans, how we process information, and, consequently, how we take decisions. AI can enhance the delivery of many goods and services, including in areas like healthcare, financial services, and technology, but it may also create bias towards a specific goal, or segment of the population, ignore it completely, or create fake news. And this is merely the beginning.
As the enormity of big data increases and computational power expands, as more data is more available to more teams and fine-tuning of Large Language Models and other AI systems becomes more affordable, we understand the technology is set to revolutionize nearly every facet of our known existence.
This big leap forward is a thrilling prospect that presents the possibility of addressing some of our most formidable challenges for the welfare of mankind. But, concurrently, it raises many valid concerns. Like any novel and rapidly developing technology, the universal integration of AI will demand a steep learning trajectory and very fast user training. Inevitable missteps and misjudgments will occur, potentially leading to unforeseen and sometimes detrimental impacts.
To optimize the advantages and curb the harm, AI ethics play a vital role in making sure that the societal and ethical ramifications of the construction and deployment of AI systems are taken into account at each phase. AI ethics rests on those 4 fundamental Ethical AI pillars and, to reinforce our message, systems need to be: Fair, Private, Robust, Explainable
Known issues so far
We are only at an inception stage in the development of AI systems, but we are all aware of some edge cases which had hit the headlines precisely because of the lack of ethical control. Here are some examples of how each pillar has been transgressed.
Fairness
The use of AI in mitigating bias in credit scores underscores why fairness is an essential factor in the engineering of AI systems. Despite often being viewed as unbiased and objective, credit scores have a lengthy past of discrimination, for instance, based on race or gender. AI-informed credit scoring models offer a more detailed data interpretation and can disclose concealed correlations between variables that might not seem pertinent or even included in a conventional credit scoring model – such as political inclinations or social media connections. However, integrating such unconventional information into credit scores risks exacerbating their existing bias. It's crucial that fairness is prioritized to ensure this supplementary data actually aids those it's intended to help, by broadening access to credit.
Privacy
The critical importance of privacy in AI usage is already evident in healthcare. In 2016, a controversy arose when London-based AI company DeepMind collaborated with the NHS, raising concerns over the handling of sensitive patient data. The subsequent move of DeepMind's health sector to its parent company, Google, fueled even more worries. It's clear that linking patient data to Google accounts cannot be tolerated. However, a suitable balance between safeguarding individual privacy and facilitating societal benefits from AI applications, such as interpreting scans or planning radiotherapy, must be struck. The true value resides in anonymized collective data rather than personal data, so a compromise should be feasible.
Robustness
The significance of robustness in an AI system is evidenced in recruitment. AI can considerably enhance the hiring process, eliminating much of the speculation involved in identifying talent and eradicating bias that frequently affects human decision-making. However, even tech giant Amazon encountered issues when their new hiring system was found to be biased against women. The system had been trained on CVs submitted over a decade, most of which came from men, reflecting the tech industry's male dominance. Consequently, the system learned to favor male candidates. AI systems aren't inherently robust – to maximize their benefits, they need to operate effectively in real-world settings.
Explainability
Microsoft's experiment in "conversational understanding" via a Twitter bot named Tay underlines why the fourth pillar of AI ethics, explainability, is necessary. It took less than a day for Twitter to taint the naive AI chatbot. The bot was supposed to improve through user interaction, learning to engage in casual and playful conversation. Unfortunately, the dialogue soon became offensive, with the bot parroting misogynistic and racist comments. Providing an explanation for an AI's decision-making is challenging, akin to explaining human intelligence, but it's vital to fully leverage the potential AI has to offer.
What does Pangeanic do to implement Ethical AI in its processes?
We believe that fair, accountable, transparent systems cannot be built on cheap labor. Pangeanic applies a fair pay policy to its suppliers, vendors, and employees. We recruit talent because it reflects on the service and the quality of products and services our clients receive.
-
Our processes are open to our clients and we evangelize them on the use of AI for their applications (machine translation, classification, data for ML, data curation...). Understanding the data and processes, we can explain how and why Pangeanic’s technology works and how decisions or output are arrived at by AI.
-
Our systems avoid bias from concept to application. Our research personnel has worked on R&D on biased publications to detect and eliminate hate speech, and gender or ethnic biases. We constantly work with our clients to create gender-balanced data sets, or data sets that are free from toxicity, for example.
-
We build, improve, customize and implement anonymization tools to respect the privacy of individuals in 26 languages. Pangeanic leads the MAPA Project, supported by the EU, and is now a core part of several anonymization infrastructures.
-
Pangeanic declares itself accountable for all its services and products in its AI and data-for-AI journey, with performance guarantees.
One very good example was the involvement of our CEO in the Translating Europe Workshop (TEW) GDPR Report, helping translation professionals to understand GDPR and how to best implement it.
After several months of engagement between representatives from translators’ associations, language services personnel, academia, and legal professionals, the group produced a recommendations report that was presented at the Translating Europe Workshop in 2022.
In the words of Melina Skondra: “Funded by the European Commission Directorate-General for Translation, the Translating Europe Workshop is the first step towards common European GDPR guidelines for the translation and interpreting profession". It encompasses the work of a panel of legal and T&I sector experts, an all-day online workshop with T&I professionals, and this summary report.
The TEW GDPR report outlines key challenges in the implementation and compliance of the GDPR in the translation and interpreting profession, explored and analyzed by the pan-European panel of experts”.
Congrats to the great team: Stefanie Bogaerts, John Anthony O'Shea LL.B, LL.M, Raisa McNab, Wojciech Woloszyk (Wołoszyk), Małgorzata Dumkiewicz, Paweł Kamocki, Zoe Milak, Manuel Herranz
Is Ethical AI the Future?
Based on fundamental rights and ethical principles, the European Commission worked on a set of Ethics Guidelines for Trustworthy Artificial Intelligence (AI). In order to build trust in human-centric AI, this political body prepared a document together with a group by the High-Level Expert Group on Artificial Intelligence (AI HLEG). This independent expert group was set up by the European Commission in June 2018, as part of the AI strategy announced earlier that year.
The Guidelines list seven key requirements that AI systems should meet in order to be trustworthy:
In an effort to put these requirements into action, the Guidelines introduce an evaluation checklist that provides advice on the practical application of each requirement. This evaluation checklist will be subjected to a testing phase, in which all stakeholders who are interested can contribute, with the aim of collecting feedback to enhance its effectiveness. Additionally, a forum has been established to facilitate the exchange of best practices for deploying Trustworthy AI.