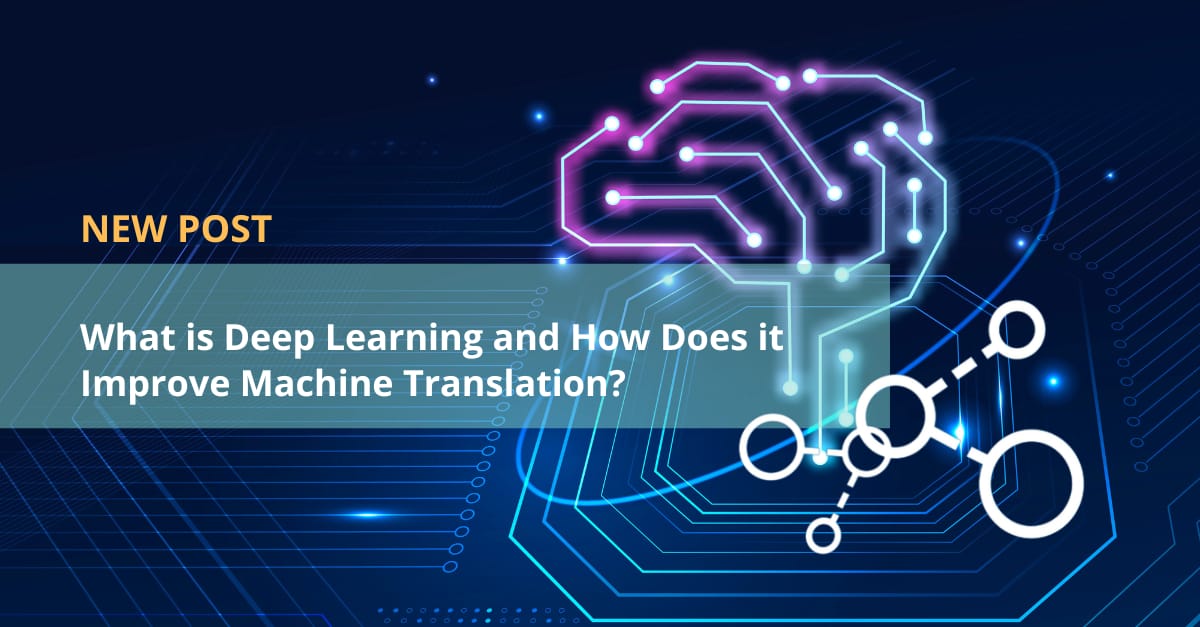
6 min read
18/05/2023
What Is Deep Learning and How Does It Improve Machine Translation?
The arrival of deep learning poses a particularly optimistic conundrum in the development of Artificial Intelligence: what if machines were capable of learning on their own, in the same way that we humans do?
Among the most exciting promises of deep learning AI is the ability to generate systems with immense predictive capabilities that can improve their performance continuously and without human intervention.
While very recently this may have sounded like a futuristic utopia, the reality is that deep learning is already part of most people's daily lives. Neural networks and deep learning are having an extraordinary impact in many different areas, from more personalized web browsing to receiving increasingly accurate medical treatments.
We will be taking a look at everything you need to know about deep learning and the different ways it is revolutionizing what machines can do.
What is deep learning and why is it important?
Deep learning is a branch of Artificial Intelligence aimed at training deep neural networks to perform complex tasks.
It is a subcategory within the broader category of machine learning that has achieved greater capabilities and flexibility. In simple terms, deep learning forms a representation of the world based on a hierarchy of concepts ranging from the most complex to the simplest and from the most abstract to the most concrete.
Thanks to its reasoning capabilities, it is now used in a multitude of ordinary applications; from speech recognition to computer vision or natural language processing, as well as machine translation.
Deep learning is bringing about very significant changes in the expectations we humans have of machines and how they work. Their importance lies in providing computational systems with the capacity to execute complex tasks with a high degree of precision, since they are capable of learning.
In turn, its value also lies in the endless automation possibilities, allowing time and money saving for complex tasks, from fraud detection in the banking sector to customer segmentation in marketing and sales.
In addition, the use of neural networks and deep learning is revolutionizing technological innovation in all kinds of industries, from medicine to manufacturing. For example, the consulting firm McKinsey recently highlighted the crucial role that machine learning pays in the area of product design.
Recommended reading:
How does deep learning work?
As we have already seen, deep learning is able to learn directly from the input data. To do so, it employs neural network architecture with multiple layers.
This operation is, at its core, based on how human brains process information. The advances in deep learning are constantly fed by developments in neuroscience, and it is the union of both disciplines that enables the generation of highly sophisticated systems.
This basic premise is embodied in the following learning process:
-
Input: at first, the data must be incorporated into the neural network (whether it be images, text, audio or any other type of data).
-
Processing: the neural network then processes the information. For this purpose, it employs multiple layers of artificial neurons, each of which performs a nonlinear transformation of the input data and then transfers the information to the next layer. The system learns as the information passes through the different layers; that is to say, it learns patterns and characteristics from the data set provided to it.
-
Output: finally, the neural network proposes a solution to the problem (a classification, a prediction, etc.) according to the desired results.
This process can be repeated over and over again, involving continuous learning that allows predictions to be made based on new data. In addition, particularly successful strategies can be implemented, such as fine-tuning which leverages a well-trained system to solve specific tasks.
Opportunities and applications for deep learning in the current climate
-
Voice recognition: systems such as Siri and Alexa use deep learning to understand and respond to user requests in an increasingly accurate way.
-
Computer vision: deep learning is used to detect objects or recognize faces from images.
-
Customer experience: the use of deep learning in chatbots has taken customer service to a new level, thanks to automation and personalization.
-
Self-driving cars: deep learning is enabling the development of autonomous driving systems, i.e., vehicles capable of making autonomous decisions in real time based on data collected by their sensors.
-
Text and image generation: machines are being trained to create text and images from scratch and automatically generate experiences such as text-to-speech. Significant breakthroughs in this area are expected based on trends such as the large language models.
-
Medicine: the use of deep learning AI is favoring more accurate disease diagnosis thanks to the analysis of medical images. Machine learning is also facilitating the development of more personalized and predictive treatments.
-
Industrial automation: deep learning is being applied to improve safety in the workplace, in addition to the generation of robots that execute tasks more precisely and are capable of adapting to different situations.
-
Finance: key areas such as fraud detection or market analysis are greatly benefiting from deep learning applications.
-
Marketing and sales: campaigns are becoming more relevant and personalized as companies are employing deep learning to analyze user behavior. This includes, for example, product recommendations on Amazon or suggested entertainment on Netflix, along with other similar cases.
The benefits of deep learning for machine translation
Machine translation systems are also undergoing a real revolution thanks to the use of neural networks and deep learning.
This is an unparalleled step forward for more advanced machine translation services that can generate highly accurate translations in a wide variety of languages, including languages that are very different from each other (such as Spanish and Chinese).
Deep learning uses text corpora to learn to translate from large amounts of text that include sentences in one language and their equivalents in another (parallel corpora).
Some areas of machine translation that have been greatly improved by deep learning include:
- Higher accuracy: systems based on neural networks and deep learning are much more accurate than other machine translation systems.
- Optimization when it comes to understanding the context, which often makes the difference between a correct translation and one that is inaccurate or ambiguous.
- Minimizing human error.
- Flexibility to adapt to different tones or text types, including technical or more informal translations.
The result is machine translation that, while requiring a post-editing service, is more fluent, natural, and accurate than ever before.
The differences between deep learning and machine learning
As explained above, deep learning is a subcategory of machine learning. However, it is still possible to establish several differences between the two concepts:
1. How they work
Conventional machine learning makes use of algorithms to extract features from a data set, from which it makes predictions, and also gives it a great abstraction capacity. On the other hand, deep learning employs neural network architectures, which allows it to learn directly from data. This, in turn, sets in motion continuous learning processes, so that the system improves its performance as its use progresses.
2. Goal
In general, deep learning focuses on identifying patterns and complex features from large amounts of data. Conventional machine learning, on the other hand, seeks to build predictive models.
3. Complexity
Deep learning involves multiple layers of information processing, as opposed to the simpler model-based operation of machine learning. In this sense, the use of deep learning AI is being able to advance at the same time that computers and their processing capabilities are advancing.
4. Type and amount of data
Machine learning works with structured and unstructured data, while deep learning uses unstructured data such as images, audio and text. In addition, deep learning requires much larger data sets.
5. Ability to establish complex patterns
Deep learning is capable of capturing complex and nonlinear patterns in data, as opposed to the simpler patterns typical of machine learning.
6. Type of training
Deep learning requires more hardware resources and more training time than conventional machine learning. However, the opposite can be said for testing, where deep learning systems require shorter test periods than most conventional machine learning systems.
7. Supervision
Conventional machine learning requires the specific supervision of programmers, who define the learning goals to reduce the complexity of the data as much as possible: thanks to the expert's guidance, the machine will recognize patterns more easily. Advances such as the zero-shot learning paradigms are, however, reducing this need.
Human supervision is reduced in deep learning processes: the system is able, to a large extent, to self-regulate and define the categories and hierarchies to be used for learning.
Data Privacy and Control in deep learning
Several dilemmas regarding the ethical use and responsibility are arising with the rapid advances in deep learning.
Experts have drawn attention to the bias that the tools could be applying, which would have a particularly significant impact when making decisions that affect individuals (e.g., during hiring processes). The lack of transparency around how a system comes to a particular conclusion can also result in problems at the ethical level when using deep learning technology.
With respect to data privacy, deep learning AI is also currently facing the following dilemma: how to exploit the full potential of the data without compromising its privacy and, in addition, comply with data protection legislation.
The reality is that training a deep learning model can raise privacy concerns if the training data contains personal or sensitive information. If finding large amounts of relevant data is already a complex task in itself, ensuring its privacy can be an additional problem.
In this regard, many tools have been developed that seek to strike a balance between the use of large amounts of data and privacy. Of note here are data anonymization techniques.
Created to manage the volume of sensitive data that companies use and store, these tools make use of natural language processing (NLP) to detect personal data in data sets and process its encryption.
The result is unidentifiable data sets that can be used in deep learning processes in a safe way and in accordance with legal requirements. These initiatives, in turn, are complemented by new approaches in this area, such as differential privacy, which implements data capture and analysis processes without compromising data subjects' right to privacy.
Regarding the challenges that arise around deep learning and privacy, it is expected that the work of thousands of specialists concerned about the advancement of this technology will end up generating a balance that includes both the benefits of deep learning and the protection of privacy and users' rights.