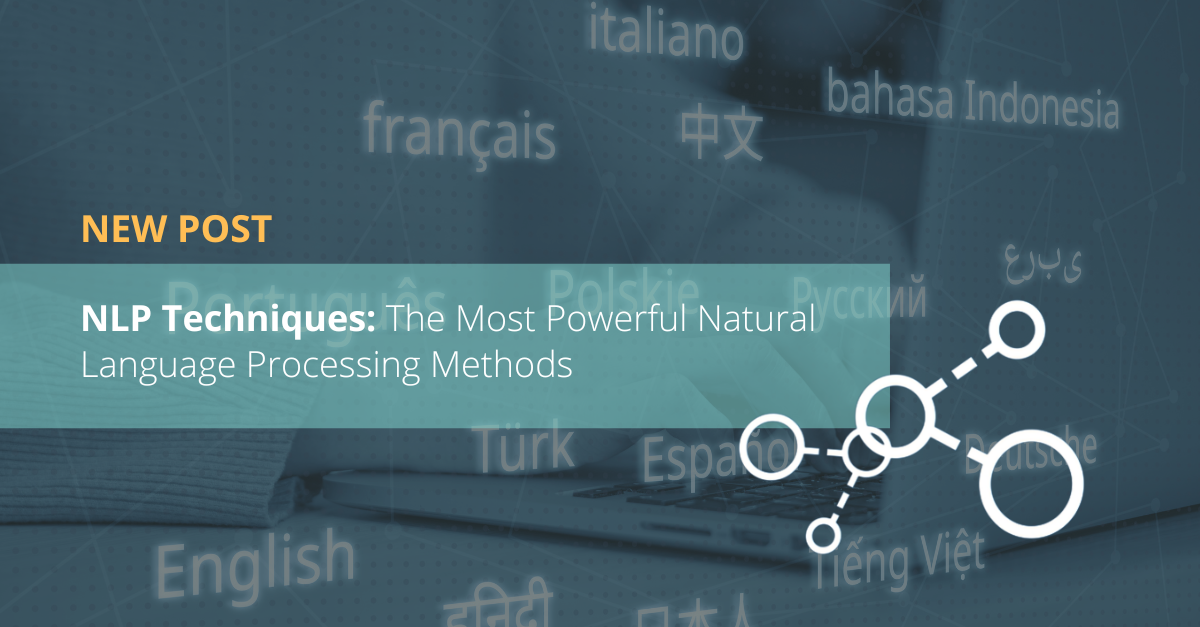
4 min read
20/03/2023
NLP Techniques: The Most Powerful Natural Language Processing Methods
In today’s NLP Techniques: The Most Powerful Natural Language Processing Methods, companies have access to large volumes of data, data that must be understood in order to deliver a better customer experience, to increase their competitive edge, to streamline internal processes, and more.
Harnessing the data and extracting the information it contains requires natural language processing (NLP) methods. More specifically, it requires the most powerful NLP techniques.
The importance of NLP techniques in the digital age
Basically, neuro-linguistic programming analyzes the way in which thought receives and interprets a stimulus and how this affects the individual’s behavior.
One of the premises of NLP is that the individual perceives the message through their senses, but according to their dominant representational system (visual, kinesthetic, or auditory).
NLP Techniques: The Most Powerful Natural Language Processing Methods, this same structure is repeated. Digital media and systems have been designed, implemented, and guided by people, therefore, NLP also applies to communication through the Internet and to the data stored by information systems. How?
Messages sent and received in e-mails, social networks, platforms, and in applications such as WhatsApp, or data produced by internal processes or on the website, NLP Techniques: The Most Powerful Natural Language Processing Methods that convey emotions. In this sense, NLP tools help to understand the internal processing of other people.
All this represents a huge amount of information for each company that must be analyzed, classified, and deciphered using the best AI-based NLP techniques so that machines can analyze every aspect of the data.
More information:
How do you know if your company needs to use NLP techniques?
If your company generates large amounts of data and captures data from third parties (customers, suppliers, competitors, partners, etc.), you need to use NLP techniques to manage the information efficiently and automate classification and analysis tasks to, for example:
-
Identify/infer topics and group texts or documents.
-
Analyze websites, online reviews, survey responses, etc.
-
Offer online recommendations on posts or products.
-
Segment audiences.
-
Detect trends.
-
Make predictions.
-
Translate technical or complex language documents.
NLP techniques for sentiment analysis
NLP methods for sentiment analysis are used to evaluate all types of text in order to detect the intent, tone, and emotion behind the message conveyed.
These methods are based on the use of tools and software that combine NLP and machine learning (areas of AI). NLP helps to execute linguistic techniques to understand text structure and recognize meaning.
Interesting content:
Application examples
These NLP techniques are often used to understand customer behavior and determine their needs or preferences. Therefore, they can be applied in:
-
Marketing campaigns.
-
Collecting and classifying feedback (positive/negative).
-
Developing products that satisfy the audience.
-
Making the right decisions to improve the customer experience.
-
Detecting specific emotions in text, among other applications.
Featured article How to boost your business with natural language processing (NLP)
NLP techniques for text classification
NLP techniques for text classification are based on deep learning methods. The latter is a subfield of machine learning that uses trained neural network models for document type recognition and differences with other texts.
These deep neural algorithms automatically classify text according to the detected content and then assign it to a category.
Application examples
This natural language technology is used in a variety of sectors, such as business, legal, finance, research, or in corporate areas such as accounting or HR. Here are some application examples:
-
Categorizing and managing business or financial documentation.
-
Pre-classifying documentation for insurance companies.
-
Evaluating business or technology trends.
-
Analyzing patents.
-
Managing information from automated support systems.
Related article:
Data anonymization NLP techniques
Anonymization is the process in which the identifying values of an individual within a data set are removed. In this way, companies guarantee the safeguarding of a person’s sensitive information, according to the GDPR, and are able to store and share information securely.
In this case, the anonymization algorithm carries out the NLP process using machine learning. In this way, it identifies and removes or replaces the different types of words (entities) that serve as identifiers within a document.
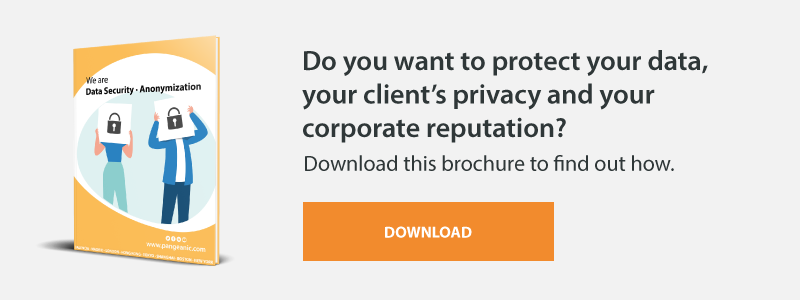
NLP techniques based on deep learning or Deep Adaptive MT models
NLP techniques that use deep learning models seek to simulate the way in which humans process information and generate knowledge.
These algorithms, also called neural networks, are stacked in a complex hierarchy in which each level of abstraction is created through the knowledge (statistical model) yielded by the previous layer. The system will perform iterations until it achieves an acceptable accurate output.
These are unsupervised methods, making them faster and more accurate than traditional machine learning.
Related article:
The use of neural networks in NLP
The most common uses of neural networks in NLP include:
-
The automatic translation of texts or spoken language.
-
Mathematical calculation using computers.
-
Speech and image recognition.
-
The generation of new text, with the correct grammar, spelling, and style.
-
Detection of satellites or troops in the military sector.
-
The automation of tasks and processes in both production and industry safety.
-
Chatbots, to improve customer management.
NLP techniques for automatic summarization
NLP techniques for automatic summarization are used to search for the most representative sentences of a document and create a summary or overview of it (extractive summarization). Data mining and machine learning are used for this purpose.
Automatic summarization can also be abstractive, using systems that summarize the text, but using context understanding and words not present in the input text. Automatic summarization can also be performed on images.
Future perspectives in the development of NLP techniques
Neuro-linguistic programming techniques are increasingly being used to make technology, such as AI, machine learning, or IoT, more natural. It is a way to make the interaction between machines and humans more widespread and eliminate the boundaries that have been in place up to now.
Thus, NLP will continue to be used to enable people to interact comfortably and warmly with intelligent systems in every aspect of their lives, for example, in systems that provide useful information or customer data management systems.
At Pangeanic, we offer high-quality language services, with NLP solutions developed with AI-based language, such as near-human-quality machine translation or anonymization, aimed at protecting the customer’s privacy.
Our solutions also feature NLP technology to categorize any type of document, as well as to achieve summarization or sentiment analysis in any language.
Do you want to achieve greater efficiency in your business? Contact us. At Pangeanic, we help you to include NLP processes in your organization.