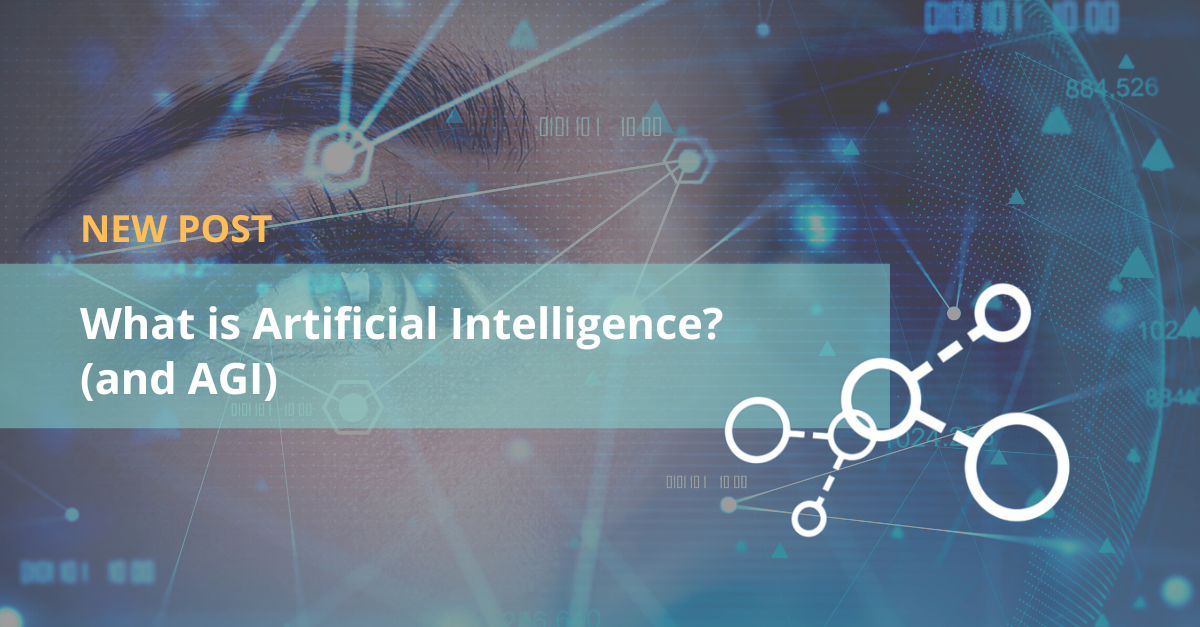
19 min read
29/11/2023
What is AI and what is Artificial General Intelligence? (AGI)
Artificial Intelligence (AI) is a transformative branch of computer science that focuses on creating intelligent machines and systems that can perform tasks typically requiring human intelligence. By undergoing training, AI systems learn from vast amounts of data, enabling them to reason, make decisions, and operate independently. Interest in this field has exploded in 2023 and continues to evolve at a tremendous speed, shaping not only the way we interact with technology, streamlining processes and unlocking new possibilities, but also the way we humans interact with each other.
In essence, AI involves software carrying out tasks with human-like abilities, including learning, perception, reasoning, and decision-making. It has become an indispensable element of modern technology, finding applications in virtual assistants, autonomous vehicles, medical diagnostics, and automated decision-making.
As we will see below, AI is already applied in multiple sectors, such as healthcare, finance, transportation, education and others, and is used to solve complex problems, automate business processes, improve customer experience and accelerate innovation.
The modern concept of Artificial Intelligence began to take shape in the 20th century. Alan Turing, a pioneer in the field and the genius who decoded the Nazis' Enigma machine, proposed the idea that machines could think. In 1948, Turing worked on the Manchester Mark I and Mark II, laying the foundations for the development of AI and designed another machine hypothetically capable of playing chess, but which failed to materialize as Torres Quevedo's "chess player", so he had to play against it using calculations of how the machine would have decided to act. Alan Turing continued working and by 1951, these prototypes were producing music. Alan Turing proposed the concept of a "Turing machine", which would be able to solve problems equal to or better than a human being.
Artificial Intelligence (AI) is a fascinating and constantly evolving discipline that is intertwined with the history of technology and computing. Our article today covers from its historical beginnings to contemporary applications, through its successes, failures and various branches, including future prospects such as Generative Artificial Intelligent (GenAI).
Types of Artificial Intelligence
Artificial Intelligence (AI) encompasses various types, and it is generally classified into two distinct categories: Narrow AI and Strong or Artificial General Intelligence. Narrow AI, is specifically designed to perform particular tasks and is increasingly prevalent in our daily lives. On the other hand, AGI represents the long-term goal of AI research: creating intelligent machines that surpass or match human abilities across a broad range of tasks. It is this strong AI that holds the potential to revolutionize the future of human-computer interaction."
Narrow AI
Artificial Narrow Intelligence (ANI) is focused on executing specific and constrained tasks. In practice, it bears a striking resemblance to the myriad of engineering and technological processes that have become familiar to us over the past decades. Virtual assistants, facial recognition, and spam filters are among the examples that exemplify narrow AI's capabilities. Importantly, these applications are primarily based on two distinct techniques.
-
Machine Learning is a crucial subtype of Artificial Narrow Intelligence (ANI). Its primary focus lies in analyzing data to enhance performance in specific tasks. Practical applications of Machine Learning can be seen in recommendation systems and chatbots.
-
Deep Learning is a specialized branch within machine learning. It utilizes artificial neural networks to extract knowledge from data, enabling models to enhance their proficiency in specific tasks. Examples of deep learning applications include image recognition and Natural Language Processing.
Artificial General Intelligence (AGI)
AGI, short for Artificial General Intelligence, stands out as the focal point of recent discussions in the realm of artificial intelligence. It often elicits concerns, yet its potential lies in possessing cognitive abilities and competencies akin to those of humans. If successfully developed, AGI would exhibit a remarkable capacity to navigate an extensive array of tasks and challenges with the flexibility and intuition characteristic of human intelligence. This encompasses everything from mathematical operations to predictive tasks and language generation.
In essence, AGI would possess the remarkable capability to autonomously learn and adapt to novel challenges and situations, without necessitating specific programming for each instance. Diverging from the limitations of weak or narrow AI, which is tailored for particular tasks like image recognition, document translation, or gaming, AGI could transcend these confines, embodying a genuine "general artificial intelligence" proficient in a broad spectrum of everyday activities requiring abstract reasoning and human-like understanding.
As of now, AGI remains an aspirational goal for entities like OpenAI, positioned in the experimental stage and representing a future frontier in the landscape of AI research
Image 1 - Star Wars movie robots, example of AI (Courtesy of Bing Image Creator)
"As a developer, I believe it won't immediately result in AGI or unsettling scenarios.”
Manuel Herranz, CEO
But why does Artificial General Intelligence (AGI) evoke such "fear"?
Essentially, the apprehension stems from the concept of embedding a generalized human intelligence into a machine. This implies that the system could potentially execute any task that a human being can perform, and do so at scale. Concerns about AGI's development revolve around whether this technology should be crafted exclusively by the handful of global companies that have the computational capacity to build it. Alternatively, there's a question of whether it should be an open-source tool, a choice that introduces its own set of challenges by providing broad accessibility to an immensely powerful technology.
The impact of AGI extends across various domains, including education and academia. Notably, the initial development of conversational artificial intelligences like ChatGPT and open-source counterparts such as META's Llama, Mistral in France, Claude, etc., did not involve any universities.
Image 2 - Logos of major AI leaders
Individuals concerned about the potential existential threat posed by AI, a founding apprehension of OpenAI, express fears regarding the emergence of rogue AI. Security becomes a focal point of worry when AI systems are granted the autonomy to set their own goals and engage with the physical or digital world.
While it is true that advancements in mathematical capabilities, such as those discussed in the Q* controversy surrounding OpenAI, may bring us closer to more powerful AI systems, solving these mathematical problems does not automatically signify the emergence of superintelligence. As a developer, I believe that it won't immediately result in AGI or unsettling scenarios. It is crucial to emphasize the nature of the specific mathematical problems that AI is designed to address.
Despite the hype surrounding these developments, they often serve more as a PR exercise, diverting attention from the tangible challenges surrounding AI. Moreover, the sensationalization of powerful new AI models can inadvertently harm the anti-regulation tech sector. Notably, the EU is on the verge of finalizing its comprehensive Artificial Intelligence Act, and one of the current debates among lawmakers revolves around whether to grant tech companies more autonomy in regulating cutting-edge AI models.
The OpenAI Board was initially conceived as an internal disconnect switch and a corporate governance mechanism aimed at averting the release of potentially harmful technologies. The recent boardroom drama underscores that, in these corporate settings, the ultimate priority often leans toward the bottom line. This development additionally complicates the argument against companies engaging in self-regulation of AI research, as it becomes increasingly challenging to make a case for the efficacy of internal controls when conflicting interests come into play.
Image 3 - Image inspired by the Alan Turing statue in Sackville Park, Manchester (Courtesy of Bing Image Creator)
As previously mentioned, AGI distinguishes itself from narrow Artificial Intelligences, such as virtual assistants or computer vision systems, which are tailored for specific tasks. AGI, on the other hand, boasts a broad spectrum of cognitive abilities encompassing real-world sensory inputs to deliver human-like responses. This may involve:
-
Natural Language Processing (NLP): The capability to comprehend (NLU) and generate text and speech (NLG) in various languages..
-
Problem Solving: The aptitude to tackle intricate problems using algorithms, logic, and reasoning.
-
Visual Recognition: The skill to identify objects, people, animals, etc., and comprehend their context.
-
Robotics: The capability to command and direct robots to perform specific tasks.
-
Decision-Making: The skill to make informed decisions based on information and evidence.
-
Social Interaction:The capacity to effectively and appropriately interact with other humans or systems, engaging in negotiations or filtering through assistants or digital twins.
-
Memory: The ability to store and leverage knowledge and experiences.
-
Creativity: Even in its early iterations, ChatGPT, Bard (now Gemini), Llama2 and then Llama3, StableDiffusion for imaging, and other distributions exhibit vast creative capabilities. These capabilities find utility in marketing, prompting-based LLM translation, summarizing information, benchmarking, and more. Consequently, creative industries may face significant impacts as they contend with the immense capacity to generate novel ideas and innovative solutions.
-
Emotional Intelligence: This involves the adeptness to recognize and manage both one's own emotions and those of others.
-
Meta-learning: This capability revolves around the skill to learn how to learn and continuously enhance one's learning process.
AGI is currently under extensive research and development by scientists and entrepreneurs globally. While a fully functional model has yet to emerge, there are ongoing projects and initiatives moving in this direction. Some notable examples include:
-
DeepMind: This Google initiative focuses on the development of a general AI capable of surpassing human performance in various board games, including Go and StarCraft. Additionally, DeepMind is exploring applications in the medical field and beyond.
-
Bard: As a language model trained by Google, Bard showcases proficiency in diverse natural language processing tasks such as text classification and response generation. Google's sustained efforts in this domain include the release of earlier versions like BERT, with a primary focus on optimizing internet search applications.
-
OpenAI: Positioned as a non-profit organization, OpenAI is committed to advancing ethical and responsible AI research and development. Among its notable projects is GPT-3, an advanced text generation model.
It's important to note that many online listings purporting to detail companies involved in AGI often refer to those engaged in narrow AI. AGI stands as a dynamic and promising field of research with the potential to significantly impact various facets of our daily lives and societal structures. Despite some hyperbolic claims, AGI remains a distant development.
Origins and Evolution of AI
AI rests on three foundational pillars: machine learning, natural language processing, and computer vision. A longstanding gap in Artificial Intelligence has been its inability to autonomously update and make instant decisions based on real-world sensor feedback. Let's briefly trace our journey to the pre-AGI era, as of the close of 2023.
Artificial Intelligence (AI) has emerged through the emulation of human intellectual processes by designing and implementing algorithms. This algorithmic approach has been pivotal in advancing AI technologies. It is essential to recognize that the journey of AI has been a collaborative endeavor, and it continues to evolve through ongoing research and development. As we approach new frontiers in AI, let us not forget the collective efforts and contributions that have brought us here.
Early Concepts and Experiments
The idea of intelligent machines can indeed be traced back to ancient mythologies, with one of the earliest known proponents being the Greek philosopher Aristotle (384-322 BC). Aristotle conceptualized a set of rules that governed certain aspects of mental functioning to attain rational conclusions.
In 1315, Mallorcan scholar Ramon Llull expanded upon the notion that reasoning could be artificially replicated in his book "Ars magna." Fast forward five centuries to 1840, and Ada Lovelace, the sole legitimate daughter of Lord Byron, envisioned machines transcending simple calculations. Lovelace provided an early conception of the software and algorithms that would drive these machines. The Spanish inventor Leonardo Torres Quevedo, born in 1852, played a pivotal role in various technological advancements. Responsible for patents related to airships used by England and France against German zeppelins, Torres Quevedo also engineered the first chess-playing machine. Unfortunately, he passed away shortly after the outbreak of the Spanish Civil War in 1936. Recognized as one of the founding figures of artificial intelligence, Torres Quevedo's legacy is honored with a dedicated PhD program in Spain bearing his name.
Image 4 - Leonardo Torres Quevedo statue (Courtesy of Bing Image Creator)
The modern concept of Artificial Intelligence really took shape in the 20th century. Alan Turing, a pioneering figure in the field and the brilliant mind behind the decoding of the Nazis' Enigma machine, introduced the radical idea that machines could possess the capacity for thought. In 1948, Turing played a pivotal role in the development of AI by working on the Manchester Mark I and Mark II, laying the foundational groundwork. He even conceptualized a machine capable of playing chess, but this vision didn't materialize, leading Turing to engage in a match against it using calculated predictions of how the machine would decide to act. Despite this setback, Turing persisted, and by 1951, the prototypes he worked on were producing music. Turing introduced the concept of a "Turing machine," designed to solve problems as well as or even better than a human being.
In the early years (1950s-1960s) of this scientific journey, the primary objectives in AI were to develop programs simulating specific human cognitive tasks, including pattern recognition and problem-solving.
A landmark moment in AI's development was the creation of the Turing test, proposed by Alan Turing in 1950. This test entails evaluating a machine's ability to exhibit intelligent behavior equivalent to that of a human. Turing posited that if a computer could communicate with a human in a manner indistinguishable from another human, it would signify the presence of artificial intelligence.
The Emergence of AI as a Discipline
However, the term "Artificial Intelligence" did not appear until the 1956s, when Dartmouth College organized an interdisciplinary meeting of students and academics, including John McCarthy, to discuss the possibility of creating computer systems capable of thinking and learning like humans. It was during this gathering that the term "Artificial Intelligence" was coined, marking the formal commencement of AI as an academic field. Both John McCarthy and Marvin Minsky played crucial roles as co-founders of MIT and, more specifically, the MIT Computer Science and Artificial Intelligence Laboratory.
The last decade saw escalating expectations across numerous sectors, with adaptive machine translation standing out as an example. This field initially experienced a moment of reassessment in the wake of the ALPAC report. Nonetheless, the 1960s proved to be a pivotal decade for AI, setting the stage for remarkable breakthroughs. It was during this period that the concept of "game theory" first surfaced, along with the development of heuristic algorithms and strategic planning. These innovations equipped machines with the capability to make rational decisions when faced with unfamiliar and ambiguous circumstances, representing a significant milestone in the evolution of their capabilities.
The emergence of expert systems during this era played a crucial role in solving complex problems. These systems were specifically designed to encapsulate the specialized knowledge and expertise of human specialists in distinct fields, thereby offering invaluable tools for addressing intricate challenges. Among the most notable achievements was the Dendral system, which demonstrated the potential of AI by successfully identifying the chemical structure of molecules based on their spectral data.
This decade set the stage for the continuous evolution of AI, with the progress made during these years acting as a catalyst for further discoveries and innovations. The developments in expert systems and machine learning algorithms laid the foundation for what would become an ever-growing and dynamic field, sparking enthusiasm for the potential of AI.
However, there have also been thwarted endeavors and unsuccessful attempts to develop systems capable of learning, retaining information, and reasoning akin to human beings—commonly referred to as "classical AI problems." AI has journeyed through waves of excitement and high expectations, only to encounter occasional setbacks due to the inherent challenges of developing such a complex and multifaceted field. Today, AI continues to thrive as a dynamic and promising domain of research, driven by the convergence of diverse disciplines and emerging technologies. Key components in this ongoing evolution include deep learning, computer vision, cognitive intelligence, and robotics, among others, each contributing to the field's vibrant growth and development."
Adolescence and the Early Stages of Artificial Intelligence
In the 1970s and 1980s, AI research underwent a shift towards creating machines with the ability to learn and adapt to new information. This era saw the development of machine learning techniques, where researchers pioneered methods for machines to learn from data and enhance their performance through experience, moving beyond reliance on predefined rules. A notable achievement during this time was the introduction of backpropagation, a method for training neural networks using gradient descent. Throughout this period, various machine learning algorithms, including k-NN, SVM, and decision trees, were conceived.
Despite these advancements, the late 1980s and early 1990s marked a phase of skepticism in AI, referred to as "the AI winter." During this period, funding and interest waned due to a perceived lack of practical results and constraints imposed by limited computing power and data availability.
However, in the early 1980s, there were breakthroughs in Natural Language Processing (NLP) techniques, rekindling interest in AI. Significantly, progress was made in human language understanding through the implementation of deep learning models and the inception of primitive neural networks. Noteworthy examples from this period include Yoshua Bengio's contributions to linguistics learning and the development of the NLTK language model.
It wasn't until the late 1990s and early 2000s that AI experienced a resurgence, propelled by the development of more robust computers, expansive data sets, and advanced algorithms. This period witnessed the ascendance of machine learning algorithms, including decision trees and support vector machines, capable of learning from vast datasets to make predictions or classify information.
Entering the 2000s, a renewed wave of interest emerged, driven by novel statistical proposals and the escalating importance of pattern recognition. An illustrative example is the release of the Moses automatic translation engine in 2011, playing a pivotal role in the creation of systems like the first version of Google Translate by Franz Och and Bing Translator by Chris Wendt. Pangeanic also entered the scene, launching its first automatic document translation platform, PangeaMT, with automatic model retraining with user data in 2011.
In the 21st century, AI is progressively weaving itself into various industries and facets of daily life. The advent of Deep Learning and the evolution of more sophisticated neural networks, such as convolutional neural networks (ConvNet) and recurrent neural networks (RNN), marked a significant leap forward in AI. These breakthroughs, key innovations since the mid-2010s, have paved the way for applications like computer vision, speech recognition, and substantial strides in machine translation. Simultaneously, there has been a resurgence in quantum AI (2010s-present), an emerging field aiming to merge quantum computing theory with artificial intelligence. Researchers anticipate that quantum AI will enable the creation of faster, more efficient learning models and facilitate solutions to complex problems in realms like medicine, physics, and cryptography.
Successes and failures
AI has undergone cycles of soaring expectations followed by "AI winters," periods marked by technological and theoretical constraints that impede its progress. The ALPAC report from the 1950s, which halted research on machine translation, illustrates how certain areas, initially dismissed as unworthy of academic attention, experienced significant delays in exploring the potential of language as a tool for AI systems.
A pivotal moment occurred in 1997 when IBM's Deep Blue defeated the world chess champion, Garry Kasparov. Despite allegations of algorithm manipulation, this event showcased AI's capability to surpass specific human abilities. However, let's delve into recent developments from 2010 to the present.
-
Adversarial Attacks: With AI becoming increasingly integrated into daily life, a new threat has emerged—adversarial attacks. These attacks involve intentionally modifying input data to deceive machine learning models, posing potential catastrophic consequences in critical applications like computer vision and robotics. Researchers are actively developing defense strategies against these attacks.
-
Explainability and Interpretability: As AI gains prominence, understanding how machine learning models operate becomes paramount. The emphasis on the explainability and interpretability of models is growing, ensuring that users trust these systems and can comprehend their decisions.
-
Ethical Considerations: AI's advancement raises crucial ethical questions about its use. Discussions encompass privacy, social justice, financial motives, and moral responsibility. Researchers and industry professionals are addressing these concerns through the formulation of policies and regulations. The EU, reflecting concerns about privacy and unregulated AI, has implemented groundbreaking regulations like the GDPR and, more recently, the AI Data Act. The United States, under President J. Biden, has responded promptly, introducing what is purportedly a stricter regulation than the European one—the "AI Bill of Rights."
Will legislation shape the future of Artificial Intelligence?
While the United States is not historically recognized for its regulatory enthusiasm, there is a discernible upswing in AI-related legislation at both the federal and state levels. At the federal echelon, the White House Office of Science and Technology Policy has outlined five guiding principles. These principles are crafted to steer the design, use, and deployment of automated systems, aiming to safeguard the American public in the era of artificial intelligence.
This initiative is driven by the objective of ensuring that automated systems, particularly those employed in sensitive domains like criminal justice, employment, education, and healthcare, are not only purpose-fit but also allow for meaningful oversight. It emphasizes the necessity of providing training for individuals interacting with these systems and underscores the importance of incorporating human considerations in instances of adverse or high-risk decisions. Furthermore, the initiative advocates for transparent public reporting on the efficacy of human governance processes.
Image 5 - Will IA help automate legal decisions? (Courtesy of Bing Image Creator)
At the state level, there's been a notable surge in proposed AI-related laws in the United States. Many states are incorporating AI regulations within broader consumer privacy laws. These regulations aim to govern AI and automated decision-making by empowering users to opt out of profiling and mandating impact assessments. Some states are also contemplating the establishment of task forces to scrutinize AI, expressing particular concern about its implications in sectors like healthcare, insurance, and employment.
The prospect of passing a comprehensive national AI law in the US within the next few years appears unlikely. Instead, the legislative focus is expected to center around less controversial and more specific measures. These may include initiatives such as funding for AI research and measures ensuring child safety in AI applications. This decentralized legislative approach could potentially lead to a mosaic of federal and state-level AI regulations.
Why is the leap to AI occurring now and not in 2015/2016 during the first wave of Artificial Intelligence startups
According to Andrew Maas, founder of several AI startups, a few years ago, business transformation was not primarily driven by executives (CTO/CIO). Fast forward to 2023, and these executives now stand at the epicenter of transformation.
Additionally, current AI technology demonstrates superior generalization across various business use cases. A few years back, customizing models was an expensive and technically demanding task. Furthermore, in 2022 and 2023, there is a proliferation of tools and technologies, offering a plethora of options for integrating AI into workflows. In the past, choices were limited, and now, there may be an abundance, with the landscape evolving every few weeks and breakthroughs happening across the board.
When selecting AI use cases, the same fundamental principles should be applied as when assessing other technologies:
-
Begin with the result (the WHAT) and work backward to the HOW.
-
Evaluate technologies with a specific group of advanced users.
-
Consider human factors, recognizing that behavior change often poses the most significant obstacle to transformation.
Currently, we lack the algorithms and architectures needed to reliably tackle mathematical problems using AI. While deep learning and Transformers, the building blocks of language models, excel at pattern recognition, this capability alone falls short of propelling AI to the level of AGI. In my capacity as a developer, aligning with the views of many academics, I emphasize that mathematics serves as a foundation for reasoning. A machine capable of reasoning about mathematics could theoretically extend its abilities to tasks reliant on existing information, such as coding or drawing key insights from a news article. Mastering mathematics poses a formidable challenge, demanding AI models to possess the capacity for true reasoning and comprehension of the subject matter. It's crucial to note that solving elementary school math problems vastly differs from pushing the boundaries of mathematical knowledge to the extent achieved by Nobel Prize-winning mathematicians.
Machine learning research has primarily centered around resolving elementary school problems; nevertheless, cutting-edge AI systems haven't entirely conquered this challenge. Some AI models struggle with simplistic math problems, while demonstrating exceptional performance on more complex ones. For instance, OpenAI has developed specialized tools capable of solving intricate problems featured in competitions for top high school math students. However, these systems only sporadically surpass human capabilities.
For a generative AI system to reliably handle mathematics, it must possess a robust understanding of specific, often abstract, concepts. Many mathematical problems also demand a level of planning across multiple steps. Notably, Yann LeCun, Meta's Chief AI Scientist, suggested on platforms like X and LinkedIn in late November that Q* is likely an "OpenAI planning attempt." Discussing this hypothesis marks a significant departure from the initial wave of AI startups circa 2015/2016, primarily focused on algorithmic problem-solving (weak AI).
If this indeed leads to AGI, a deeper grasp of mathematics could unlock applications aiding scientific research and engineering. The capability to generate mathematical solutions could enhance personalized tutoring or assist mathematicians in quicker algebraic problem-solving and tackling more intricate issues.
This isn't the first instance where a new model triggers AGI speculation. In 2022, similar assertions were made about Google DeepMind's Gato, a versatile AI model proficient in playing Atari video games, providing captions for images, engaging in chat, and manipulating physical objects with a robotic arm. Some AI researchers at that time contended that DeepMind was on the brink of AGI due to Gato's diverse skill set. However, in reality, it was another episode of the same hype, just unfolding in a different AI laboratory.
Artificial Intelligence has traversed a considerable distance from its initial concepts to emerge as a transformative influence in contemporary society. Amidst its successes and setbacks, AI exhibits remarkable potential to enhance our lives and challenge our perceptions of machines' capabilities. Looking ahead, AI will not only progress in technical capabilities but will also grapple with substantial ethical and social challenges. GenAI, applied to text, image, or sound generation, signifies a new frontier in this evolution, opening avenues for creative and technical possibilities. As a society, we must be poised to steer this evolution responsibly, ensuring that AI contributes positively to humanity as a whole.
Industries Impacted by Artificial Intelligence
No sector, excluding manual labor, remains immune to the influence of artificial intelligence. Even traditionally manual occupations like taxi driving, goods delivery, or shopping for food can be subject to automation, either entirely or in part. The ramifications of this extend into the realms of employment, labor dynamics, and the dynamics of human interaction.
Image 6- Self-driving taxi (Courtesy of Bing Image Creator)
Artificial intelligence (AI) finds application across a diverse range of sectors, serving as an invaluable tool for enhancing work processes and boosting productivity. However, the automation of jobs and the subsequent workforce reduction is a multifaceted issue with implications for many industries. Some companies, particularly those heavily reliant on knowledge management, translation, or programming, have already begun downsizing, merely a year after the introduction of ChatGPT.
Initially, AI can lead to the elimination of jobs that precede its implementation, notably in the manufacturing sector, where it has the potential to replace traditional factories with more advanced automation systems. Nevertheless, AI also has the capacity to create new employment opportunities, as seen in the systems engineering sector, where skilled personnel are essential for developing and maintaining these systems.
Moreover, the application of AI can enhance productivity and efficiency in existing jobs, enabling individuals to complete larger or more complex projects within the same timeframe or with fewer resources. This advancement might result in increased production capacity for companies without the need to expand their workforce, or alternatively, a smaller team of highly skilled workers could accomplish the tasks previously handled by a larger staff.
Furthermore, automating jobs with AI empowers professional employees to leverage their skills and resources for the development of new roles and responsibilities. This can encompass roles related to learning, the exploration of new technologies, or the monitoring of systems.
FINANCIAL SECTOR
In the financial sector, artificial intelligence is reshaping banking and finance through informed decision-making, trend prediction, and fraud detection. Examples of AI applications in this sector include:
-
Risk Analysis: AI can analyze vast volumes of financial data to evaluate customers' credit risk, facilitating well-informed lending decisions.
-
Fraud Detection: AI plays a crucial role in identifying suspicious transactions, mitigating potential financial losses.
-
Personal Financial Assistants: AI aids users in managing their personal finances, offering insights for making informed investment and savings decisions.
TRANSPORTATION SECTOR
Artificial intelligence is reshaping transportation through the implementation of autonomous driving, traffic management, and urban planning. AI is already optimizing routes and logistics operations, potentially replacing jobs related to manual routing and scheduling. Companies utilizing AI-powered systems can cut costs, enhance efficiency by minimizing empty kilometers, and optimize fuel consumption.
Examples of AI applications in the transportation sector include:
-
Autonomous driving: AI controls autonomous vehicles, reducing accidents and traffic congestion.
-
Traffic management: AI analyzes data from sensors and cameras to manage traffic flow, minimizing delays.
-
Urban planning: AI aids urban planners in designing efficient and sustainable cities, using algorithms to determine optimal building, street, and park placement.
ENERGY SECTOR
Artificial intelligence is revolutionizing energy management, optimizing production, transmission, and consumption. Examples of AI applications in the energy sector include:
-
Renewable energy production: AI optimizes solar and wind energy production by predicting weather conditions and adjusting production accordingly.
-
Electrical grid management: AI monitors and controls the electrical grid, ensuring efficient and safe power distribution.
-
Energy efficiency: AI assists consumers in reducing energy consumption by providing personalized recommendations for saving energy in homes and businesses.
EDUCATION SECTOR
Artificial intelligence has already transformed education, enabling personalized learning, enhanced feedback, and pattern identification. Examples of AI applications in education include:
-
Content recommendation systems: AI analyzes student behavior patterns and interests to recommend personalized educational content.
-
Virtual tutoring: AI-based virtual tutors offer instant and personalized feedback to students, improving academic performance.
-
Data analysis: AI analyzes large amounts of student data to identify success patterns and enhance teaching.
PRODUCTION AND MANUFACTURING SECTOR
Artificial intelligence is revolutionizing production and manufacturing through process automation, efficiency improvement, and cost reduction. While AI can replace manufacturing jobs through automation, it can also create new roles, such as maintaining and operating automated systems.
Examples of AI applications in production and manufacturing include:
-
Process automation: AI automates repetitive tasks like manufacturing, assembly, and product inspection.
-
Failure prediction: AI analyzes data from sensors and equipment to predict possible failures and prevent downtime.
-
Computer-Aided design: AI aids designers in creating innovative and efficient products by generating optimized designs.
HEALTHCARE INDUSTRY
Artificial intelligence is transforming healthcare by enabling early disease detection, improving diagnostics, supporting personalized medicine, and automating administrative tasks. While certain jobs involving repetitive tasks may be replaced, AI enhances healthcare services, allowing professionals to focus on critical areas.
Examples of AI applications in healthcare include:
-
Medical diagnosis: AI analyzes extensive medical data to help doctors diagnose diseases and predict test results.
-
Treatment personalization: AI assists doctors in tailoring treatments for patients based on individual characteristics and medical history.
-
Patient monitoring: AI monitors patients with chronic diseases, notifying doctors of any changes in their condition.
AI is also revolutionizing audiovisual content production and consumption. For instance, the streaming giant Netflix employs machine learning algorithms to suggest movies and TV shows based on user viewing history and preferences of similar users.
These examples showcase how artificial intelligence is applied across various sectors. AI's potential extends to transforming numerous industries, enhancing efficiency, productivity, and user experiences in diverse contexts.
Risks and Impacts of Artificial Intelligence:
As AI continues to impact society significantly, it's crucial to consider ethical and social aspects related to its development and usage. Some ethical challenges include:
-
Risk of bias and discrimination: Machine learning algorithms may perpetuate biases and discriminations present in society, widening the digital divide and perpetuating injustices
-
Data privacy and security: Large-scale collection and analysis of personal data pose risks to privacy and increase the likelihood of security breaches and identity theft.
-
Accountability and transparency: Establishing responsibility for AI system decisions and ensuring transparent decision-making processes are crucial ethical considerations.
-
Job impact: Automation and AI-driven job replacement can negatively affect certain professions and economic sectors, necessitating continuous adaptation and training.
-
Security and stability: AI can be exploited for malicious activities like hacking or spreading false propaganda, posing threats to the security and stability of society.
Addressing these challenges requires a clear regulatory framework ensuring responsible and ethical AI use. Investing in education and training for AI skills is equally essential to prepare all stakeholders for the forthcoming changes.
Ethical Challenges of AI
AI introduces numerous ethical challenges demanding attention to ensure its development and use benefit society. Some of these challenges include:
-
Discrimination: AI systems may exhibit bias if trained with data reflecting social biases, leading to challenges like facial recognition systems struggling to identify people of color when trained on predominantly white population data.
-
Privacy: AI systems relying on personal data collection pose risks to privacy as they process information to enhance performance.
-
Safety: AI systems are susceptible to cyber attacks, posing risks of data theft, system disruption, or even physical damage.
In conclusion, while AI is a potent tool capable of transforming various aspects of daily life, addressing the ethical and social challenges tied to its development and use is imperative. This ensures a positive impact that benefits society as a whole, given the increasing influence of AI on our daily lives.