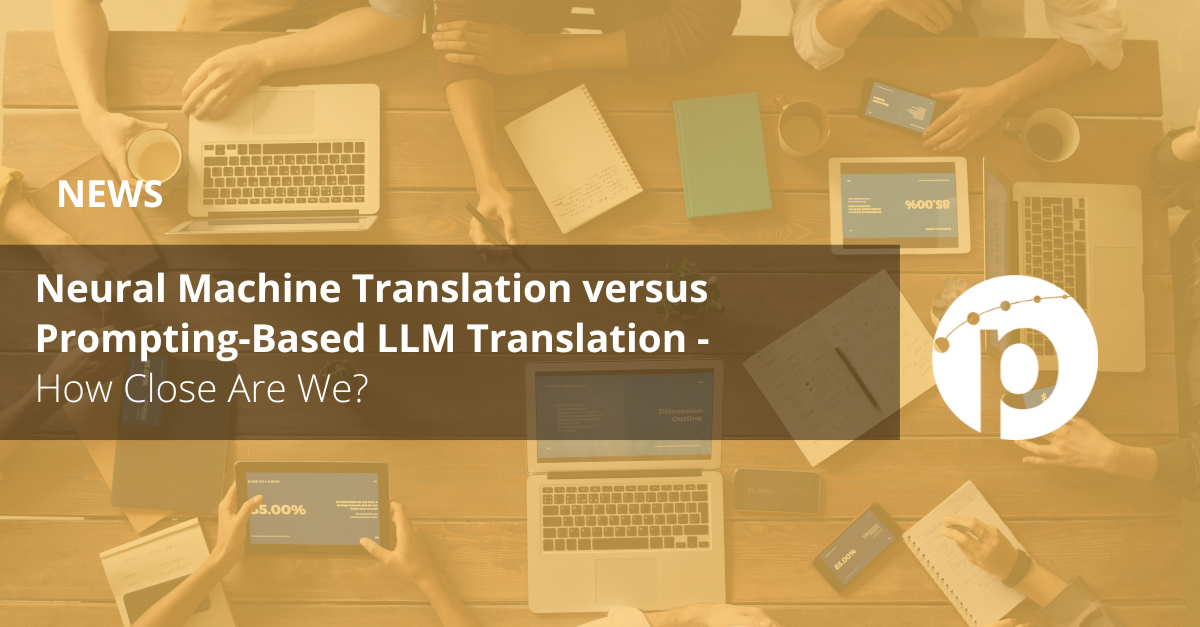
7 min read
18/05/2024
Neural Machine Translation versus Prompting-Based LLM Translation - How Close Are We?
The recent GALA Association annual conference in Valencia has officially kick-started the conference season. And of course, the dominant issue (the only issue, I would say) was AI - or rather GenAI and how it is affecting the language industry, whether there will be a language industry at all in a few years, the role and value of humans in a world where AI can produce fluent content as good as humans.
I summarize separately Pangeanic's contribution to the conference and my participation in three presentations on "Ethics of AI in the translation industry," chaired by my admired Olga Beregovaya of Smartling, our Use Case with news agency EFE, and "Walking the Path of Resistance to Use GenAI in Production" with my also admired Kirti Vashee of Translated.
The localization industry has experienced a transition from bilingual corpora-trained models to glossaries, adaptive MT, and now prompting-based MT using large language models (LLMs). Neural Machine Translation (NMT) remains popular due to its flexibility, scalability, and cost-effectiveness.
In 2024, the best approach to customized MT continues to be neural MT. I I will not put my money where we will be in 2025, not even at the end of 2024. However, Adaptive Machine Translation (NMT) is still the fastest and most economical machine translation solution for most applications and the gold standard. It is undoubtedly the easiest technology to control and adapt. Those are just several reasons for it. I am not the only one defending the validity of the technology: Christian Federmann from Microsoft has always defended the flexibility of NMT models.
Why Adaptive (Neural) Machine Translation Reigns
There are four main reasons for this and the panel at "Walking the Path of Resistance" round table (Kirti Vashee, Jose Palomares, and Helena Moniz) gradually dealt with them.
1. Economic Scalability: NMT has reached a level of maturity where the availability of parallel corpora allows for the creation of MT engines at significantly lower costs. This makes NMT an economically viable option for many organizations, whether on-premises or on the cloud, enabling corporations to deploy high-quality MT solutions without exorbitant expenses.
2. Adaptability: NMT models can be customized swiftly by injecting data into a baseline model with various levels of adaptation (we call it aggressivity as the process is automated by an algorithm). At Pangeanic, for instance, we can customize models in minutes through different levels of parallel corpora injection, ensuring that the translation output aligns closely with specific client requirements. This adaptability is crucial in maintaining the relevance and accuracy of translations across different domains. The adapted engines produce exactly the content users want.
3. Controlled Output: NMT excels in delivering controlled output, making it ideal for specific domains such as e-commerce, software, healthcare, and even subtitling - full of idiomatic nuances. Maintaining consistent terminology and style is critical in these fields. There can be many cases of conflicting terminology between those fields, so maintaining separate engines makes complete sense. Despite GenAI's impressive capabilities, controlling the terminology and style is essential for enterprise users. NMT's ability to adhere to predefined glossaries and style guides ensures that translations meet the required standards of accuracy and coherence.
4. Human Verification: A professional workflow in NMT involves human verification of TMX files before injecting them into adaptive NMT engines for re-training. This step is essential to ensure the customized model produces high-quality translations that meet the client's standards. Human oversight acts as a safeguard against potential errors and inconsistencies, enhancing the overall reliability of the MT system.
Prompting-Based LLM Translation
Prompting-based translation is proving very popular. However, there are advantages and disadvantages. The largest disadvantage, for me, is the lack of control over the output (and I’m speaking after our experience after customizing our ECO LLM 8x7B model for translation).
Let’s not forget that LLMs are GenAI (Generative AI), modeled to be generative. We are used to having the same results in science and engineering if we apply the same formula. We all know that asking the same question to an LLM does not necessarily guarantee the same translation result. That may not be a bad thing for the occasional user if you’re a bureau, office, or small business or if you just need to translate an email. I cannot stop being surprised at how fluent our ECO LLM is at translating from Japanese or Arabic into French, Catalan, or Spanish, for example – much more fluent than Google! So the question is Why are we not using prompt-based translation at scale?
Challenges of Prompting-Based LLM Translation
Many MT and TMS companies are integrating Generative AI into their workflows. This integration offers exciting possibilities, but it also comes with challenges. According to McKinsey: "The honeymoon phase of generative AI (gen AI) is over. As most organizations are learning, it is relatively easy to build gee-whiz gen AI pilots, but turning them into at-scale capabilities is another story. The difficulty in making that leap goes a long way to explaining why just 11 percent of companies have adopted gen AI at scale."
![]()
|
![]()
|
A view of ECO NMT Arabic to English translation versus Prompting-Based LLM translation by ECO LLM
I chose a translation into English so most people can judge the subtle differences.
كما يناقشان كيفية مواجهة الرؤساء التنفيذيين للتحديات الجيوسياسية المتغيرة، وتأثير الذكاء الاصطناعي التوليدي داخل المؤسسات، وكيفية الانتقال نحو استخدام الطاقة المستدامة، بالإضافة إلى ذلك، نستعرض في عدد هذا الشهر مجموعة من الموضوعات البارزة الأخرى التي تدور حول: كيف تستطيع المؤسسات في مجال التصنيع الاستفادة من الإمكانات الهائلة لتقنيات الذكاء الاصطناعي. دور المدراء الماليون المهم في تحويل استراتيجيات الشركات من أجل تعزيز قيمتها وخلق فائدة أكبر لها. استراتيجيات وتقنيات يمكن لمديري عمليات الاستحواذ على صناديق الأسهم الخاصة استخدامها لزيادة القيمة التشغيلية للشركات التي يستحوذون عليها. أولويات الرؤساء التنفيذيون لتحقيق التميز والريادة خلال فترة قيادتهم. |
They also discuss how CEOs face changing geopolitical challenges, and the impact of generative AI within organizations, How to move towards sustainable energy use, In addition, In this month's issue, we will cover a range of other high-profile topics that revolve around: How can manufacturing organizations take advantage of the enormous potential of AI technologies? The important role of financial managers in transforming corporate strategies in order to enhance their value and create greater utility for them. Strategies and techniques that private equity fund acquisition managers can use to increase the operational value of the companies they acquire. CEO priorities for achieving excellence and leadership during their leadership period. |
كما يناقشان كيفية مواجهة الرؤساء التنفيذيين للتحديات الجيوسياسية المتغيرة، وتأثير الذكاء الاصطناعي التوليدي داخل المؤسسات، وكيفية الانتقال نحو استخدام الطاقة المستدامة، بالإضافة إلى ذلك، نستعرض في عدد هذا الشهر مجموعة من الموضوعات البارزة الأخرى التي تدور حول: كيف تستطيع المؤسسات في مجال التصنيع الاستفادة من الإمكانات الهائلة لتقنيات الذكاء الاصطناعي. دور المدراء الماليون المهم في تحويل استراتيجيات الشركات من أجل تعزيز قيمتها وخلق فائدة أكبر لها. استراتيجيات وتقنيات يمكن لمديري عمليات الاستحواذ على صناديق الأسهم الخاصة استخدامها لزيادة القيمة التشغيلية للشركات التي يستحوذون عليها. أولويات الرؤساء التنفيذيون لتحقيق التميز والريادة خلال فترة قيادتهم |
As they discuss how to address the changing geopolitical challenges, the impact of generative AI within institutions, and the transition towards sustainable energy, we will also explore other prominent topics in this month's issue, including: How manufacturing companies can leverage the immense opportunities provided by AI technologies. The crucial role of financial managers in translating corporate strategies to enhance a company's value and create greater benefits for it. Strategies and techniques that corporate raiders can use to increase the operational value of the companies they take over. Priorities of executives to achieve distinction and leadership during their tenure. |
This is precisely the crossroads the translation industry finds itself. While it is relatively simple to use ChatGPT for translation, it becomes much more challenging to do so at scale when several other factors are considered. GenAI, despite its potential for higher automation, does not always guarantee the customization and consistency required for professional translations. Companies are experimenting with GenAI, but its implementation is still evolving. Here are some drawbacks (but don't worry - the advantages follow):
1. Lack of Control: One of the most significant challenges with prompting-based LLM translation is the lack of control over the output. Unlike NMT, which provides consistent results when the same inputs are used, LLMs, being Generative AI, can produce varying translations for identical inputs for no reason. This unpredictability can be problematic for large-scale translation projects requiring terminology consistency.
2. Generative Nature: LLMs are designed to be generative, which means they can create varied outputs that might not always align with the required terminology and style guidelines. For example, in professional translation tasks that demand strict adherence to specific terminologies and consistent style, LLMs may struggle to deliver the desired consistency.
3. Use Cases: While LLMs excel in generating fluent translations for casual or small-scale needs, their effectiveness diminishes for large-scale, consistent translations. They are suitable for translating emails, casual conversations, or one-off documents but may not perform well when precise terminology and style consistency are crucial. Ensuring consistency segment after segment is a challenge: the LLM seems to have a mind of its own, getting bored of repetitive tasks. For no particular reason, it can suddenly begin to “generate”.
The potential of LLM-Based Translation
Implementing machine translation technology has always required a keen understanding of the starting point (translation memory-based workflows) and the final objective. The same applies to moving from neural MT to LLM-based translation. We need to be aware of current capabilities and future potential. Dual use may be an option, leveraging the strengths of NMT while exploring the possibilities of GenAI. The localization industry, not known for its forward-looking adoption of many technologies, must continue to deliver high-quality, customized translations that meet the evolving demands of global communication.
True, the approach of prompting-based machine translation using large language models (LLMs) offers more natural and contextually relevant translations than NMT, mainly when domain-specific training data is limited or non-existent. LLM translation is excellent for Japanese <> Spanish or Polish <> Mandarin, off-the-cuff. I see the value here (no longer pivoting through English and obtaining culturally-relevant translations).
The point is… how long will we hold to NMT? Not so long, I dare say. The push and the investment budgets are on private GenAI, despite that 11% of all PoC and projects become successful implementations, according to McKinsey. So I do envisage GenAI systems that, at a similar or higher cost, offer a lot more automation from a single API connection, benefitting from GenAI’s fluency and post-editing in context at scale.
For specialized tasks, custom-trained LLMs can deliver high-quality translations. However, achieving this requires sophisticated prompting techniques, and tested workflows, and often, significant computational resources. Without these, LLMs may produce free-form translations that lack the precision and accuracy required by professional translators.
Future Outlook: Shifting from NMT to Prompting-based LLM translation
Like it or not, the industry may gradually shift from NMT to GenAI as its capabilities improve. GenAI promises higher levels of automation, contextual fluency, and the ability to handle complex translation tasks from a single API connection. From my point of view, the ability to provide contextual fluency is the single most important point when starting to think about adopting prompting-based LLM translation.
Conclusion
The future of customized machine translation is bright, with NMT and GenAI offering unique advantages. While NMT currently leads in control, consistency, and cost-effectiveness, GenAI holds promise for more natural and contextually relevant translations. As the industry continues to innovate, the balance between NMT and GenAI will shape the future of professional translation services. By staying informed about these technologies and their evolving capabilities, translation professionals can make informed decisions that best meet their clients' needs.
Are you thinking of transitioning from your current NMT to GenAI? Walk the walk with Pangeanic. Contact us to explore how we are implementing GenAI-based automatic post-editing at News Corporation EFE.