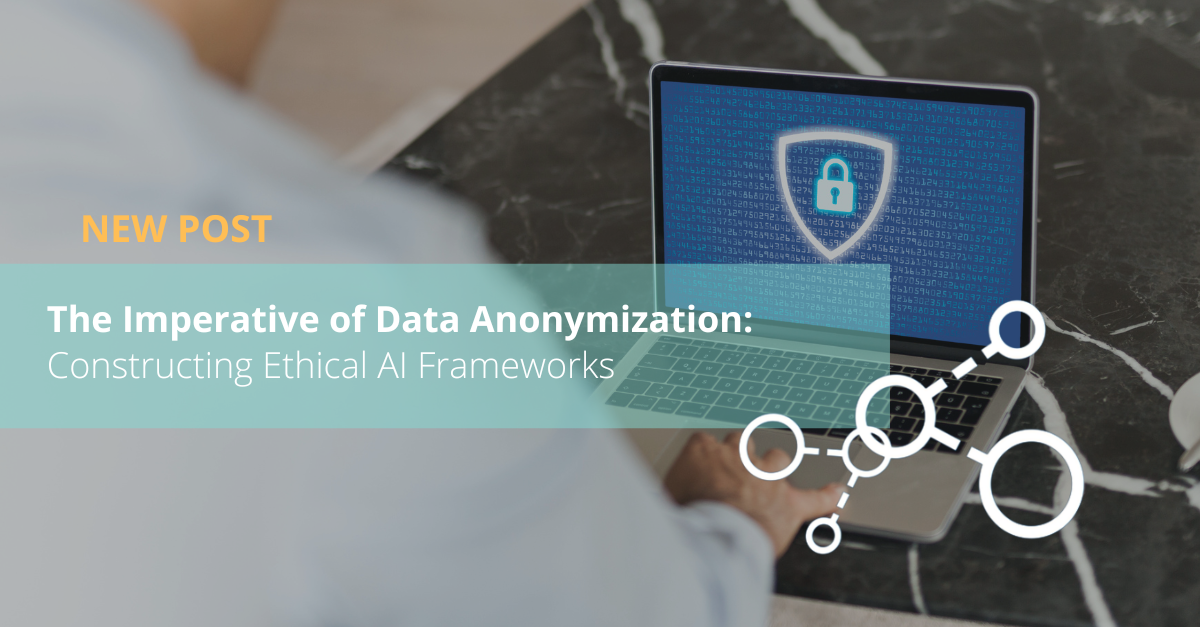
3 min read
11/07/2023
The Imperative of Data Anonymization: Constructing Ethical AI Frameworks
We live in the age of data-driven decision-making. Data has become the new oil, and personal data is one of the most refined fuels. As a result, artificial intelligence (AI) has emerged as a transformative force, profoundly influencing every sector from healthcare to finance and beyond.
The power of AI comes with an inherent responsibility — ensuring the ethical use of the data it relies upon. At the heart of this ethical obligation lies the concept of data anonymization, a critical yet often overlooked aspect of constructing ethical AI frameworks.
Data anonymization refers to the process of shielding identifiable data so that individual privacy is preserved while the data remains useful for analysis. This de-identification process is crucial for not just complying with evolving data protection regulations but also for ensuring that the development and implementation of AI systems are ethical.
Recommended reading:
Anonymization Regulations, Privacy Acts and Confidentiality Agreements
Navigating the Intersection of Privacy and Utility
The sophistication of AI algorithms today means that an unprecedented amount of data is collected, stored, processed, and analyzed. While this data is instrumental in improving and tailoring AI systems, it raises substantial privacy concerns. These concerns become amplified when AI systems handle sensitive data such as healthcare records, personal identification information, or financial transactions. It's at this intersection of utility and privacy that data anonymization becomes a fundamental pillar in constructing ethical AI.
Anonymization techniques such as pseudonymization, aggregation, or encryption not only safeguard privacy by reducing the risk of personal data exposure but also maintain the data's usefulness for AI algorithms. They ensure the rights and freedoms of data subjects without hindering the development and deployment of AI systems. The more data needs to be gathered, collected, and shared across systems, the higher the risk PII is at stake.
We, users/consumers/citizens may think there’s nothing we can do about it and that privacy is no more in times of smartphones, geolocation, social networks, etc. However, custodians of our data cannot act freely. There are and must be regulations that limit the uses of personal data in AI training or directly enforce its removal — basically because we don’t know where our personal data may end up and who can query it.
The Legal Perspective: Data Protection Regulations
From a legal standpoint, the importance of data anonymization cannot be overstated. Data protection laws, such as the General Data Protection Regulation (GDPR) in the European Union or the California Consumer Privacy Act (CCPA) in the United States, impose strict rules on how personal data can be processed. Non-compliance can lead to significant fines and penalties.
Under these laws, anonymized data is not considered personal data. Therefore, if data anonymization is executed correctly, businesses can sidestep the complexities of these regulations and avoid the severe consequences of non-compliance.
It’s crucial to understand that the standards for what constitutes effective anonymization are high, especially given the capabilities of modern machine learning algorithms to potentially re-identify anonymized data. Hence, governments and businesses must keep abreast of both technological advancements and legal developments to ensure their anonymization techniques are legally compliant and ethically responsible.
Trust in AI: The Role of Data Anonymization
Trust is the bedrock of AI adoption. With escalating concerns about data privacy and misuse, it's clear that trust hinges on demonstrating a commitment to data privacy and ethical AI development. Proper data anonymization practices foster this trust, enhancing transparency and credibility in the eyes of customers and stakeholders alike. Moreover, it can give companies a competitive edge, especially as consumers and businesses become more discerning about how their data is used.
You may be interested:
A Temporary Pause in AI Development: Is Artificial Intelligence Arousing Fear Among Experts?
Anonymization as an Ethical Imperative
Anonymization is not just a legal necessity but also an ethical imperative. Pangeanic embarked on a data masking/anonymization journey five years ago when the European Commission approved its proposal to develop a multilingual, open-source anonymization software (MAPA Project). Since then, we have championed the concept of Ethical AI not only in fair pay to developers but also in the use of personal data in AI training.
The development of AI systems must be guided by a steadfast commitment to the principles of privacy, transparency, and fairness. Data anonymization provides a practical and effective means of honoring these principles.
By incorporating robust data anonymization processes, we can ensure that AI realizes its full potential in a manner that respects individual privacy rights and adheres to ethical standards. It’s a challenging balance to strike, but one that is crucial for the sustainable and responsible growth of AI technology.
From Pangeanic, we have developed our anonymization tool: Masker.
Masker harnesses the power of AI to automatically detect personally identifiable information (PII) and substitute sensitive data with various anonymization techniques. Tailor the level of sensitivity and select specific tags to personalize the anonymization process according to your needs.
- With ID tags, data is replaced by generic data tags (e.g., ORG1, NAME2, ADDRESS3).
- Substitution replaces data with temporary identifiers (e.g., ACME, JOE, 123 Somewhere St.).
- Gaps replace data with empty spaces.
- Redaction replaces data with solid black lines.
These Data Masking methods are meticulously designed to minimize the risk of tracing data or electronic trails that could lead to misuse or disclosure of personal information.
Here is an example of Redaction Data Anonymization.