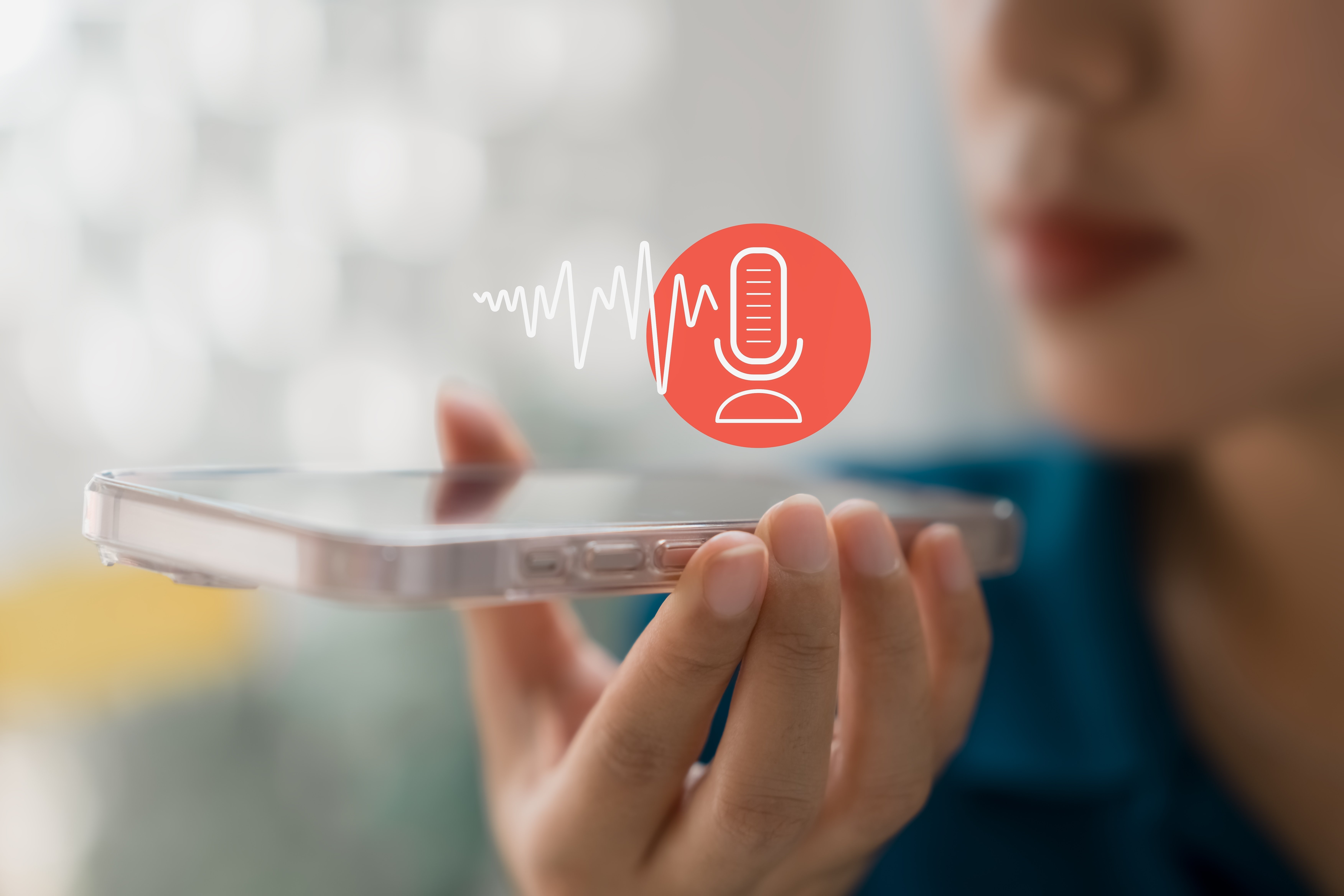
3 min read
15/05/2025
Comprehensive Voice Data Processing for AI: A Flagship Project
In Artificial Intelligence systems, data quality is paramount. Poor training data—even in small proportions—can have devastating effects on a model’s final performance. Developers of all sizes understand this well: "noise" in machine learning training sets results in models that are unreliable in their decisions.
Building more accurate models, achieving more reliable outcomes, and developing more responsible technologies can only stem from data that is thoroughly cleaned, well-processed, and meticulously annotated. This is in addition to advanced techniques such as Reinforcement Learning with Human Feedback (RLHF). Pangeanic has established itself as a leading provider of Data-for-AI services, offering end-to-end data solutions for training Artificial Intelligence models. These solutions combine cutting-edge technology with highly skilled human expertise. Our comprehensive approach and proprietary PECAT platform are fundamental to projects that require the careful handling of large volumes of multilingual and multicultural data. We integrate diverse disciplines to deliver sophisticated, high-quality outcomes for the next generation of AI models.
We would like to share with our readers one of Pangeanic’s recent projects as a clear example of this methodology in action.
The Challenge: 2,000 Hours of Raw Audio in Multiple Languages
A major international client entrusted Pangeanic with the processing of over 2,000 hours of raw audio recordings in a variety of languages and formats (WAV, MP3, FLAC, among others). These recordings spanned multiple use cases—including scripted speech, spontaneous conversations, and call center interactions—and presented significant challenges: variable quality, background noise, and inconsistent metadata.
In short, the data was unrefined. And as mentioned earlier, an AI model is only as good—and as broad—as the data it is trained on. The first and most critical step, therefore, was the precise preprocessing and segmentation of the audio files.
1. Preprocessing and Segmentation with Timestamps
Pangeanic’s team began by segmenting each audio file according to the client’s specifications. This involved identifying and timestamping each relevant segment, classifying them by language, domain, audio quality, and other technical parameters. This stage was essential to transform chaotic raw data into organized training material, ready for ingestion by the client’s algorithms.
2. Data Ingestion and Management via PECAT
Once preprocessed, the audio data was imported into PECAT, Pangeanic’s proprietary data annotation platform. PECAT—short for Platform for Efficient Content Annotation and Tagging—enables the management of complex annotation projects online, in real time. It combines technical oversight with expert human intervention, ensuring seamless task assignment, quality validation, and uninterrupted workflow.
3. Human Transcription and Linguistic Enrichment
A cornerstone of this project was the manual transcription of audio files. Here, Pangeanic deployed its extensive network of specialized linguists and transcription professionals. Thanks to their expertise, the transcriptions achieved a level of accuracy and consistency tailored to each language and dialectal variation, surpassing the current limitations of many automated systems.
4. Speaker Diarization and Turn Annotation
For each audio segment, speaker identification was performed, indicating conversational turn-taking. This process is crucial in conversational recordings or call center audio, where it is necessary to determine which content belongs to which speaker.
5. Named Entity Recognition (NER)
The team then carried out Named Entity Recognition (NER), a key step in training linguistic models. Entities such as personal names, organizations, locations, and dates were identified and annotated in accordance with the client’s guidelines.
6. Personal Data Anonymization (PII)
To ensure compliance with privacy regulations, Pangeanic implemented anonymization of personally identifiable information (PII). This involved both tagging and, when necessary, modifying or masking the original audio to guarantee that no sensitive information remained exposed.
7. Metadata Enrichment
Finally, each file was enriched with comprehensive metadata, facilitating its future use in AI engines. All relevant details—such as language, domain, duration, speaker count, and audio quality—were compiled using standardized formats.
Final Deliverable: JSON and Custom Formats
The project concluded with the delivery of a complete data package in JSON format, along with any other formats required by the client. Each audio file had been processed, annotated, transcribed, anonymized, and enriched. In just four weeks, Pangeanic completed the full data treatment cycle, delivering a high-quality dataset ready to power AI model training.
Technology, Platform, and Human Expertise: Pangeanic’s Comprehensive Approach
This project serves as a clear demonstration of how the synergy between proprietary technology (PECAT), standardized processes, and specialized human talent enables Pangeanic to offer end-to-end data solutions. From preprocessing to final delivery, every phase was overseen by expert teams, following a "human-in-the-loop" approach that ensures quality, accuracy, and ethical compliance.
In a world where AI increasingly relies on reliable, clean, and ethically sourced data, Pangeanic reaffirms its commitment as a global technology partner, capable of scaling and tailoring solutions for multilingual, multicultural, and multi-domain projects. After all, artificial intelligence is only as effective as the data that feeds it, and no one understands this better than Pangeanic.
Would you like to learn more about how Pangeanic can help transform your data into AI value?
Visit www.pangeanic.com and discover everything our technology and talent can do for your projects.