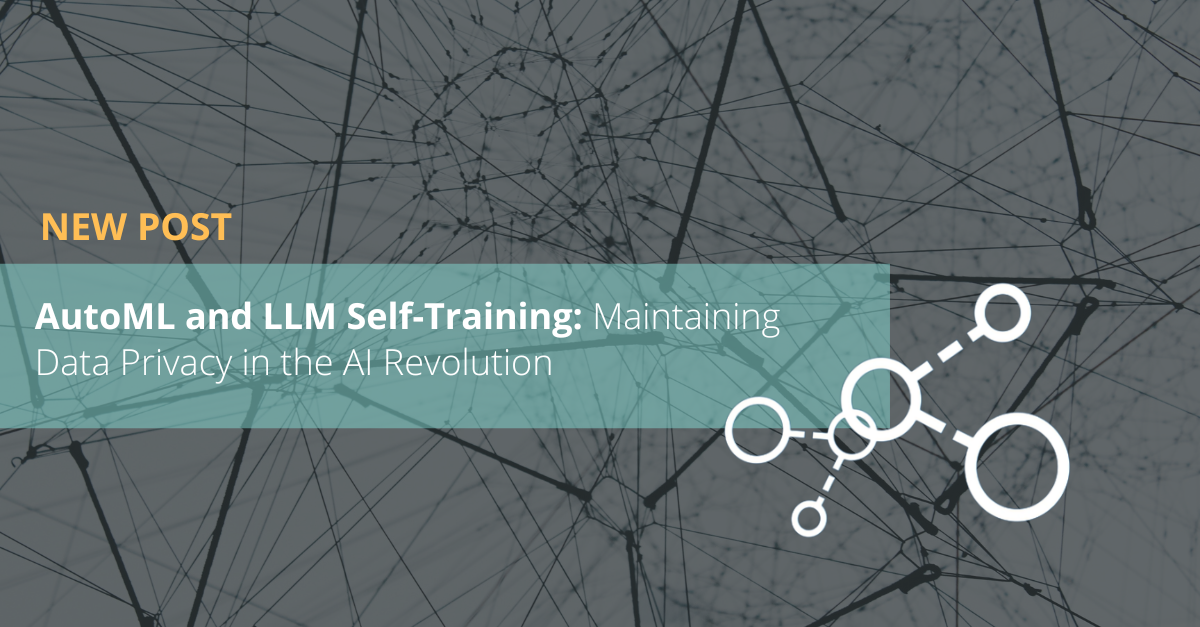
3 min read
26/07/2023
AutoML and LLM Self-Training: Maintaining Data Privacy in the AI Revolution
Data is the fuel powering the AI revolution. However, organizations face a growing challenge: sharing their data with large tech giants for AI model training often means compromising the privacy of their users or sensitive business information. In this context, AutoML (Automated Machine Learning) and LLM (Large Language Models) self-training have emerged as powerful alternatives.
This article will delve into the advantages of these methods that allow organizations to embrace AI benefits without sharing their data with external entities.
Defining AutoML and LLM Self-Training
-
AutoML refers to the automated process of end-to-end development of machine learning models. It involves automating data preprocessing, feature selection, model selection, and hyperparameter tuning. It allows users with varying levels of expertise to develop machine-learning models with high efficiency and minimal manual intervention.
-
Large Language Models (LLMs) are advanced AI models trained on vast amounts of text data. They can generate human-like text and understand context, making them valuable tools for numerous applications, such as chatbots, content creation, and more.
Self-training LLMs refer to the process where organizations train these models on their specific datasets without sharing data with external parties.
Advantages of AutoML and LLM Self-Training
1. Enhanced Data Privacy
By employing AutoML and self-training LLMs, organizations can maintain full control over their data. They don't need to send sensitive information to third parties for model development, significantly reducing the risk of data breaches or misuse. This approach ensures data privacy regulations compliance, a crucial aspect in sectors like healthcare, finance, and education, where stringent data privacy rules apply.
2. Customized AI Solutions
Running AutoML and self-training LLMs allows organizations to develop AI solutions tailored to their specific needs. Unlike generic AI models offered by large tech giants, these bespoke models can address unique business challenges and contribute to more effective decision-making. They can learn the nuances of an organization's data, enhancing the performance and relevancy of the generated insights.
3. Reduced Dependency
Self-training of AI models reduces the dependency on external tech giants, providing more autonomy to organizations. It allows them to control the timeline of their projects, modifications to the AI model, and its deployment, without having to rely on third-party timeliness or availability.
4. Intellectual Property Protection
Using AutoML and LLM self-training, businesses can protect their intellectual property. The insights derived from their data, the AI models developed, and the related technological advancements remain in-house, giving them a competitive edge.
Recommended reading:
5. Cost-Effective
While there are costs associated with setting up AutoML and LLM self-training processes, in the long run, these methods can prove cost-effective. Organizations don't have to pay for the use of third-party AI services continually. Instead, they can invest in creating a robust AI infrastructure that will serve them over time.
6. Flexibility and scalability
With AutoML and self-training LLMs, companies can flexibly adapt their AI models to new requirements or changing business conditions. They are not bound by fixed models or parameters imposed by external service providers. In addition, these methods offer high scalability. As the amount and variety of data increases, the models can be continuously adapted and optimized to deliver better and better results.
7. Faster implementation and innovation
AutoML and self-training of LLMs can lead to faster innovation and implementation. Instead of waiting for external vendors to develop and deliver models, companies can develop and customize their AI solutions internally. This not only speeds up solution delivery but also fosters a culture of innovation as organizations can continuously improve and re-train their models.
8. Better comprehension and trust
By leveraging AutoML and self-training LLMs, organizations maintain control over their AI processes and models. This not only promotes a better understanding of underlying mechanisms and predictions but also confidence in AI solutions. At a time when understanding and being able to explain AI decisions is becoming increasingly important, this can be a key advantage.
Conclusion
In the age of data-driven decision-making, the ability to use and control data effectively while maintaining its privacy is a significant competitive advantage. AutoML and self-training LLMs offer an opportunity for organizations to embrace the benefits of AI while maintaining control over their data.
The initial investment and learning curve can be steep, but the long-term benefits – customized AI solutions, enhanced data privacy, reduced dependency on tech giants, intellectual property protection, and cost-effectiveness – make it a worthy consideration.
In a world where data is becoming increasingly valuable, the combination of AutoML and LLM self-training offers organizations the ability to use data efficiently and securely. While some investment and learning are required to adopt these methods, the benefits in terms of data protection, customized solutions, independence, intellectual property protection, and cost-effectiveness make them an attractive choice for many organizations.
These methods represent an exciting new direction in AI development that allows organizations to maintain control and take advantage of technology to drive their affairs and be masters of their own future.