What is Neural Machine Transaltion? Neural Machine Translation (NMT) is the new approach to machine translation. NMT works with an end-to-end...
The combination of human and artificial intelligence remains the key to achieving excellent results in natural language processing. Despite this, machine translation is increasingly present thanks to its ability to process huge amounts of data, making it a powerful and crucial tool for breaking down language barriers.
However, the key to achieving this balance between technology and manual translation lies in the post-editor. The human part in charge not only of ensuring the quality of translations, but also of training artificial intelligence models to bring us closer and closer to the ambitious human parity.
Today, we talk to
MªÁngeles García Escrivà, Senior Project Manager at Pangeanic,
to find out about the human-in-the-loop figure from the inside.
Machine translation is often a topic that divides opinions in the translation industry; why do you think this is?
On the one hand, many linguists fear they will be out of a job if machine translation gets too far. On the other hand, customers fear that they will receive a translation of inferior quality if it is done by a machine.Could you explain to us what the human-in-the-loop concept is and how it applies to your experience as a linguist and translator?
In the case of machine translation, human-in-the-loop refers to human participation in its development and how their contribution is essential in various aspects of the process. We could name, as a main aspect, the role of the translator in the post-editing task that offers human quality to the final client based on a machine translation, which speeds up the workflow. Humans' contribution (linguists, in this case) in the translation and revision of data used to train the artificial intelligence models used in machine translation models should also be mentioned. And we cannot forget that none of this would be possible without the work of the humans who create the machines and train them, by which I mean engineers, developers and scientists, of course.Regarding machine translation engines' learning, we know that Pangeanic works with different languages from around the world. What are, from your experience as a translator, the most complex ones?
Continuing with the above example, it is not easy to get documents translated from Icelandic to Chinese, or vice versa, unless they are provided upon request, which is costly and sometimes almost impossible, as it is very difficult to find human translators with that language combination. For this reason, the best performing engines tend to be those with the highest number of available translated documents.
You may be interested in: The latest trends in the translation industry
Which ones do you think pose the greatest challenge to the machine and the human translator?
I definitely don't think there are complex languages, the human translator knows their native language well, regardless of whether it is Chinese, Zulu or Spanish. They will have no problem translating if they also have a good knowledge of the text's source language and domain. However, in the case of the machine, the most influential factors are the amount and diversity of data available for training, and the level of belonging to the domain of the text to be translated. Therefore, coming back to the same case as before, it will be languages that do not have large amounts of translated data; this situation normally occurs with minority languages ( languages used by a small number of speakers in a community or State).Let's talk about ECO. Some readers may think that the emergence of such platforms threatens the role of the human translator. What do you think about the matter?
The huge advances in AI in recent times will lead to the disappearance of many types of professions as we know them today, but will lead to the emergence of new, much-needed positions in the imminent future.What is the role of the human translator in relation to machine translation engines?
Right now they have 2 main functions:- The “post-editor” (a figure introduced in the linguistics sector when machine translation first appeared) is responsible for correcting any errors in the machine translation results, and for improving the style and appropriateness of the target text.
- The validator and creator of translation units to be used for the training of machine translation engines. This role requires knowledge of linguistics and translation and is an essential role for training machine translation engines.
What benefits do you think having tools like ECO brings to the human translator?
The most notable benefit of ECO, I would say, is the time saved during the translation process. Just as computer-assisted translation tools have already done, translation engines allow the translator to translate in a more timely manner by providing part of the translation that will be used by the translators in the target text.Can you tell us about your experience of a project where human and artificial intelligence were combined?
As I mentioned before, projects that include post-editing in their workflow would be a good example, since, on the one hand, the machine provides the basis on which the translated text will be worked on and, on the other hand, the human perfects the text to achieve an adequate document for the target audience, taking into account whether the text is technical, legal, academic, etc. Keep in mind that the same term may belong to different fields or domains and, depending on which domain it belongs to, its translation may be different. Moreover, even within the same domain, it is possible that the translation of a term may be different depending on the context. For example, there are different Spanish translations for the English word “control” in the field of medicine, as in the example “to control a hemorrhage”, the correct translation being “ detener una hemorragia ”, and not “ controlar una hemorragia ”. Another good example is the term “ birth control pill” (“ píldora anticonceptiva ”) as the translation of “control” here is included in the word “ anticonceptiva ”. Today, there are specialized translation engines capable of making these distinctions, but not all of them do, and especially not in all language combinations. Therefore, in these cases, it is even more necessary to have the help of a human to check that the translation is appropriate to the domain and context in question.
Keep learning → What is human parity in machine translation?
Regarding machine translation, can you give us a prediction for the future? What is in store for future generations of translators and linguists?
Translation will probably become even more specialized. Translators will need to be experts in certain fields. In the years to come, machine translation will need large quantities of translated documents to train engines, especially specialized documents that teach the machine to identify those distinctions that make the difference between a common term and a specialized term. And not simply specialized, but specialized in a certain field and context.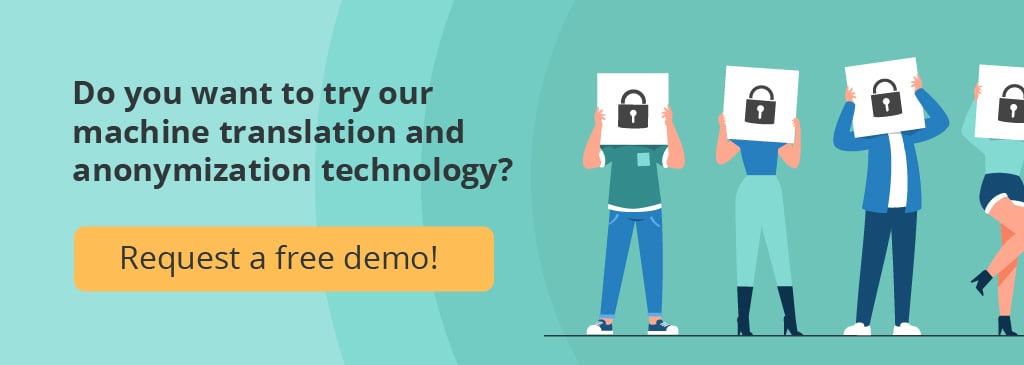
Related Articles
There are over 7,000 languages in the world – some allow for easy machine translation, while others present a major challenge for these translation...
Translating the same content into different languages, known as multilingual translation, is a challenging endeavor. Some of the difficulties that...