At the SwissText & KONVENS 2020 conference from June 23-25, 2020, Pangeanic presented the Multilingual Anonymisation toolkit for Public...
INEA has awarded Pangeanic's consortium almost €1M to develop a multilingual anonymization toolkit based on AI processing of health, life science, and legal texts for Public Administrations. The MAPA Project (Multilingual Anonymisation toolkit for Public Administrations) will make use of state-of-the-art Natural Language Processing tools to develop the open source toolkit with a focus on the medical and legal domains, deploying it at several Public Administrations in Europe.
"The aim of MAPA is to provide data anonymization so language data can be shared across and between organizations, while protecting private or sensitive data. Implementation cases will focus on de-identifying, obfuscating or pseudo-anonymizing personally identifiable information to prove not matter which language a Public Administration or user deals with, the solution can cope. MAPA will enable PA's to comply with GDPR to a high degree of accuracy and protect an individual’s private details while maintaining the usefulness of the source data." - Manuel Herranz, CEO
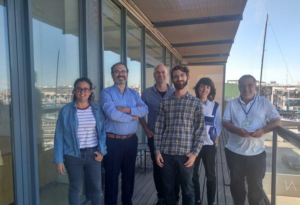
Why Anonymize Data?
GDPR obliges organizations to protect citizens' data so it is not released to 3rd parties (see this video on Pangeanic's anonymization technologies). The MAPA data anonymization toolkit will provide the means to share language data while protecting personal or sensitive data. Being able to release large amounts of anonymized data can help the community to have more training data. On a more practical level, justice departments, health authorities, healthcare companies will be able to provide access to data and manage an anonymization strategy. Most importantly, MAPA will satisfy GDPR requirements at scale. Although no software can guarantee 100% accuracy in anonymization, just as perfect machine translation does not exist (yet), it will make document sharing much easier.Technical Approach to Anonymization
At its core, the MAPA anonymisation toolkit will use Named-Entity Recognition and Classification (NERC) techniques using both Deep Learning techniques and neural networks. In addition, thanks to the transfer learning capabilities shown by new types of Deep-Learning models, new systems can be trained using relatively small datasets of manually labelled data. The knowledge acquired for a given domain or language can be transferred and re-used cross-language or cross-domain. MAPA will be trained to detect named entities that involve sensitive information. MAPA will be feature-rich and the NERC approach will be complemented with other configurable mechanisms such as pattern detection based on regular expressions (passport or ID numbers, telephone numbers, street addresses, blood groups, age, sex, marital status, email addresses, bank accounts, etc.) User-definable dictionaries for particular applications will also cater for specific usages of entity names known in advance.Use cases
MAPA includes several specific deployments/use cases for public institutions at several EU countries: one for the health domain and one for the legal domain. Both domains were selected given their strong anonymization requirements prior to any publication and sharing of the data. In each deployment case, the system will be tailored to the specific needs of the relevant institution.MAPA is funded by the Connecting Europe Facility (CEF) programme, under grant No A2019/1927065, and will run from January 2020 until December 2021.
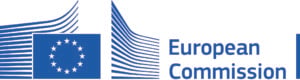